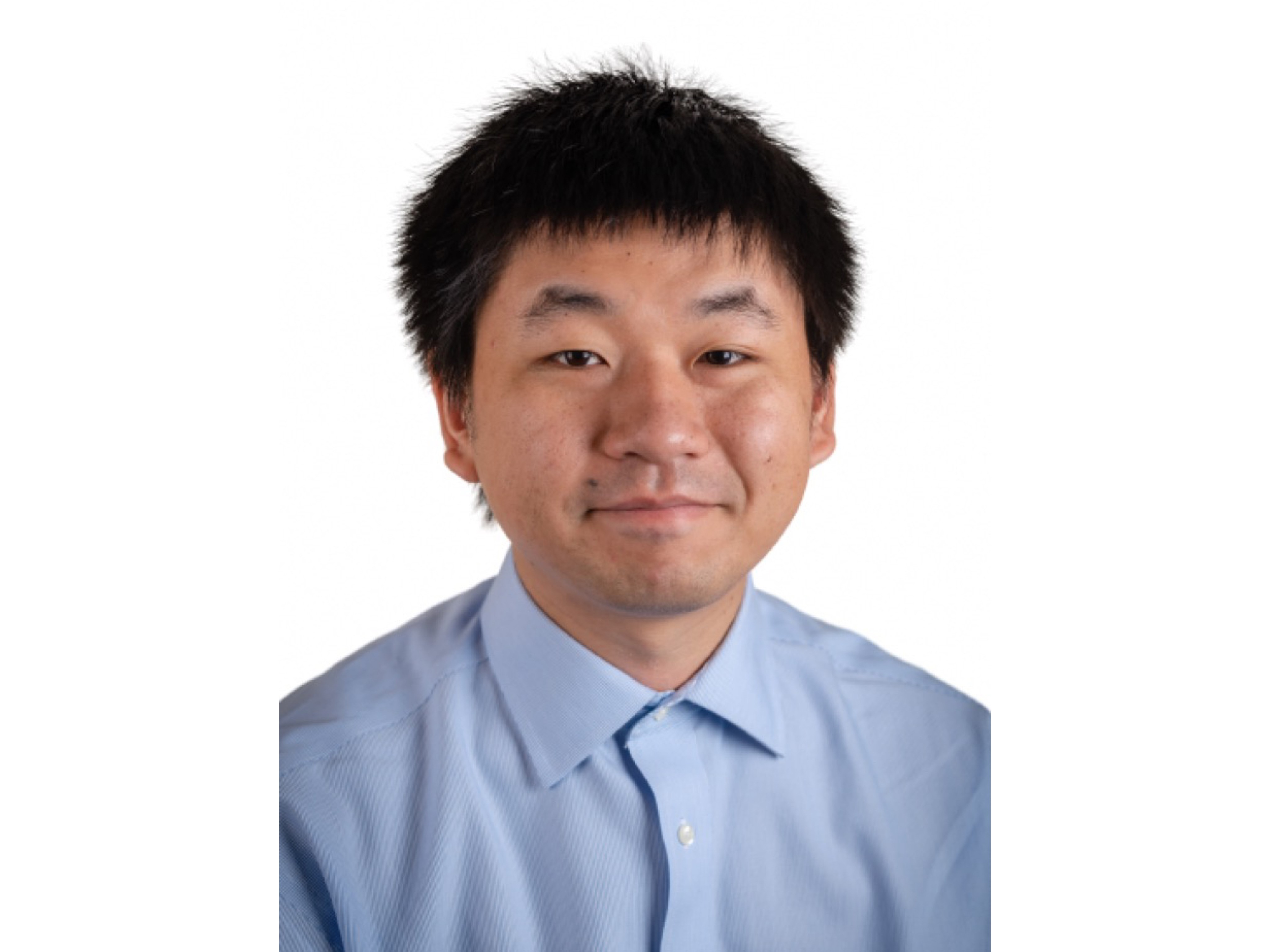
By Jovina Ang
SMU Office of Research & Tech Transfer– There is a trend across the world showing that healthcare systems are moving towards the adoption of capitation models to limit excessive healthcare costs, improve patient health outcomes and reduce the performance of unnecessary patient services.
In Singapore, the healthcare system will start to move progressively to this model from April 2023.
Capitation models are based on a capitated contract by a health insurance company and a medical provider.
The models follow a fixed, pre-arranged payment received by a physician, clinic or hospital per patient enrolled in a healthcare plan or per capita. The agreed amount is normally based on local costs and the utilisation of services.
Given that payments are pre-determined and fixed, the models hope to deter healthcare providers from ordering more procedures or provide more services than necessary.
However, in practice, there is evidence indicating that the capitation models do not work well.
Based on the studies of capitation models in the United States, it has been shown that healthcare providers tend to cherry pick the more profitable patients to provide services for. In other words, risk selection exists in the capitation models.
Assistant Professor of Operations Management, She Zhaowei conveyed the intent of his research to the Office of Research & Tech Transfer: “Given that big data analytics have revolutionised work, especially in transforming terabytes of data into actionable insights, I wanted to investigate if big data and machine learning could be the cure to solving the risk selection problem in capitation models.”
“To probe into the matter, I decided to focus on the Medicare Advantage (MA) programme, which is the largest capitation model in the United States. In this capitation model, a flat fee or a risk adjusted rate per patient is paid regardless of the amount of services provided,” he continued.
“In the research, I wanted to investigate and understand the root causes of risk selection. I also wanted to know if big data can eliminate risk selection especially in perfectly providing availability of information with data showing the exact amount for treating each patient type”, he added.
The research
Professor She partnered with Turgay Ayer, Associate Professor at Georgia Tech and Daniel Montanera, Assistant Professor at Grand Valley State University to design a game theoretical analysis model for the research project.
The project relied on big data analytics and machine learning. Many steps were taken for the researchers to construct a game theoretical analysis model that mimics the scenarios that might take place in the real world.
The research team also compared how the model would work in traditional Medicare where the fee paid would vary depending on the service provided, to the capitation model where a fixed fee would be paid per capita of patients.
Additionally, the team simulated a scenario where there would be perfectly available information to understand the actual amount of fees for treating the different patients.
The other focus areas of the research included a deep dive into the causes of risk selection behaviours, as well as a thorough analysis on the risk selection that is induced by cross subsidisation of healthcare incentives.
Insights from the research
What the research shows: A lack of perfectly available information is not the only cause of a failure in the capitation models.
Even if big data is used to provide an environment where there is perfectly available information, the issue of risk selection would still persist.
While advancements in big data and machine learning could address the problems of risk selection, the issue of cross subsidisation would still be ignored in the capitation models.
It is simply not feasible for a generic machine learning algorithm to take into account the underlying mechanisms and, possibly, the different health plan incentives for treating the different patient types as different capitation payments are used for the different sub-groups of patients.
This explains why risk selection that is induced by cross subsidisation would persist.
Implications of research
Given the increased adoption of capitation models, this research has application and interest to payers, providers and policy makers in the healthcare market.
A key takeaway that Professor She would like to leave behind is: “Big data is not the panacea of risk selection in capitation models”.
“Risk selection can be induced from the subsidisation of subgroups of patients using capitation payments collected from other subgroups. This is an important point that payers, providers and policy makers need to take note of,” he said.
Back to Research@SMU May 2022 Issue
See More News
Want to see more of SMU Research?
Sign up for Research@SMU e-newslettter to know more about our research and research-related events!
If you would like to remove yourself from all our mailing list, please visit https://eservices.smu.edu.sg/internet/DNC/Default.aspx