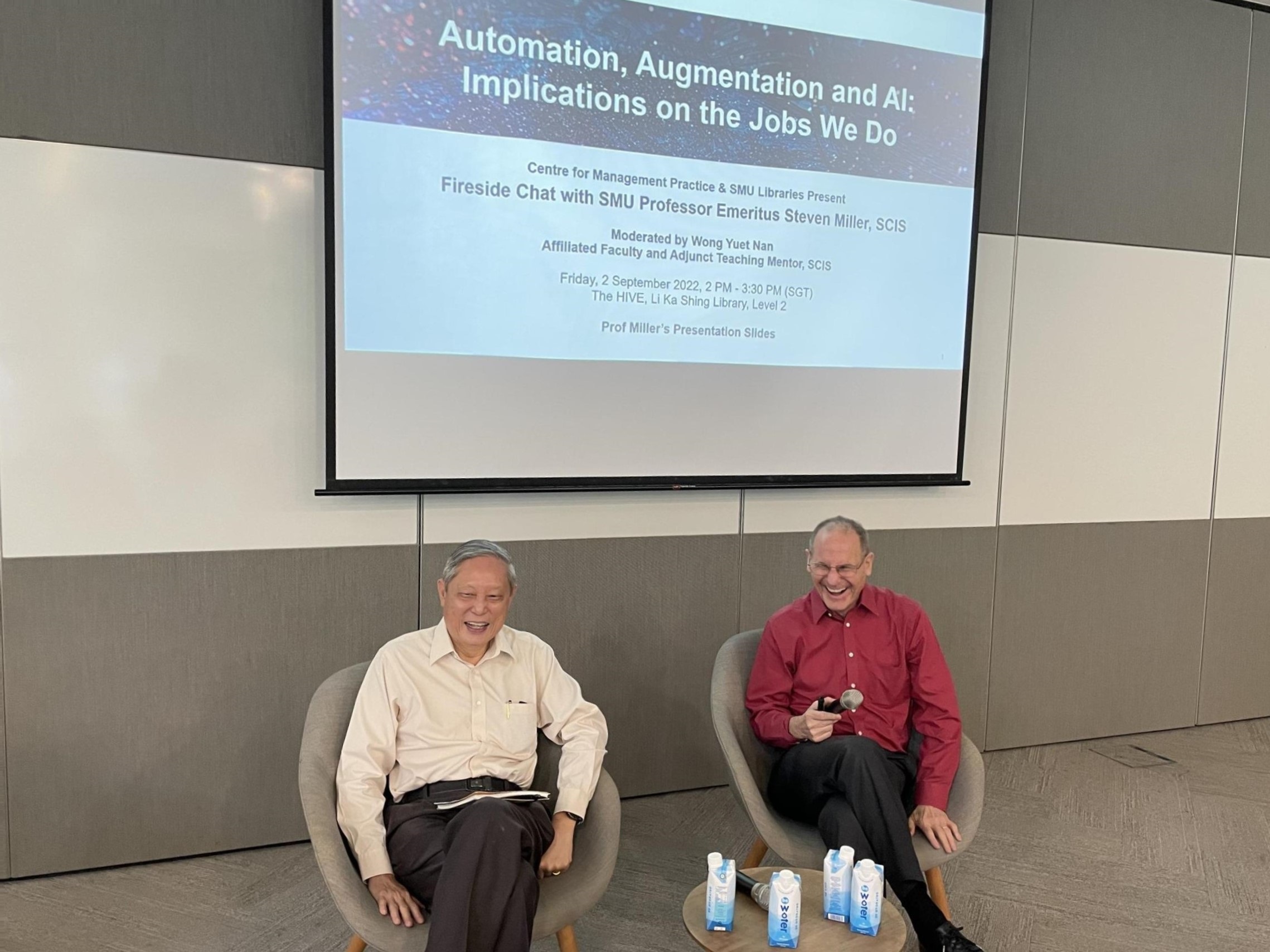
By Alvin Lee
A new generation of AI-enabled machine capabilities
SMU Office of Research & Tech Transfer – Humans have always been creating new ways of using tools to improve their ability to survive, work, and amuse themselves. Eventually, this led to the ability to create machines that could fully automate certain types of human work. Since the usage of automated machines in the 1500s and until very recently, the essential requirements for being able to automate what a person does was the ability to explicitly codify all knowledge required to do every task required to complete a particular human job, and the corresponding ability to create hardware and/or software machines to replicate that codified knowledge and execute the required actions (physical or cognitive).
Relatively recent developments in the sub-field of AI referred to as machine learning over the past ten years have changed this situation.
Now, by providing a machine learning algorithm with enough examples – enough data on the inputs and outputs of a single task or set of tasks – the algorithm can detect complex and many-dimensional patterns that enable it to infer and often accurately predict how to perform the various tasks. Hence, it is now possible to use machine learning – often in combination with other AI methods – to perform tasks that were previously impractical or nearly impossible for humans to completely codify accurately and robustly such as recognising an image, translating language, generating conversational or written text, or even doing some types of scientific discovery such as predicting how a protein will fold into a 3-dimensional shape. These new AI-enabled capabilities, largely driven by developments in the ability to apply various types of machine learning methods (e.g., neural networks, reinforcement learning, graph learning, clustering and classification methods, and others) have greatly extended the range of what is technically possible and economically feasible to do by machine.
As these technology trends continue and as more companies begin to make use of machine learning capabilities and embed them into their portfolio of digital transformation initiatives, this naturally raises questions about the role of humans in the current as well as future workforce. One immediate question is whether there will be an even greater degree of displacement of human employees by machines going forward as a result of the new generation of AI-enabled automation that has a wider range of applicability than prior generations of automation.
Automation andaugmentation
These were topics explored at the recent Fireside Chat that was organised by the Centre of Management Practice and SMU Libraries titled “Automation, Augmentation and AI: Implications on the Jobs we do” that featured a presentation and Q&A with SMU Professor Emeritus of Information Systems Steven Miller. This session, held on 2 September, was hosted by SMU SCIS adjunct faculty member Wong Yuet Nan. Professor Miller co-authored a book with Thomas Davenport titled, “Working with AI: Real Stories of Human Machine Collaboration” that was released by MIT Press at the end of September. Professor Miller drew from the 29 case examples in this book as well as from nearly 30 other AI deployments that he has studied over the past three years. This article is based on that session as well as on follow up discussions with Professor Miller.
Professor Miller explained that automation is not the only way that AI and other workplace technologies are put to use. He clarified that economists use the term ‘automation’ in a specific way to refer to situations where technology – including AI-enabled information systems and physical machines – is used as a substitute for the human worker and as a result, the worker is displaced from that job which is automated. That worker might be redeployed within the organisation to do other work or might be retrenched.
An alternative way of using technology is for augmentation where, for example, AI-based tools in the form of software systems or machines are used to complement and often amplify the capabilities of the person doing the job. This enables the person doing the job to work more productively and sometimes even be able to do things that were not possible to do previously without the support of the AI-enabled augmentation. Rather than displacing a person via automation, augmenting an employee’s job usually increases the quality and quantity of their output and therefore increases the employee’s contribution (and therefore value) to the employer.
A spreadsheet is a well-known example of a modern information management tool that has been used to augment the work of many employees across a wide range of occupations. Today’s AI-enabled capabilities are resulting in a new generation of “supertools” that can complement and amplify a human worker’s capabilities far beyond what a spreadsheet can do. And for that matter, AI methods can be embedded in spreadsheets and in many other types of existing information systems commonly used in today’s workplaces.
One of Professor Miller’s key points was not to jump to the conclusion that increasing usage of AI-enabled technologies in the workplace will always lead to automation and job displacement. Of course, sometimes it will, though in fact all of the deployment examples he has recently observed while writing the book and through his other studies involved augmentation. He referred to a local example of using AI systems to support the operations and monitoring of the Mass Rapid Transit (MRT) urban rail network in Singapore.
“The rail network operations staff constantly monitor all six MRT lines,” he explains. “Automated sensor systems feed data into an AI-enabled analysis platform call FASTER and automatically generate predictions of impending disturbances such as, ‘Two minutes from now, there will be a slow-down due to a malfunction of a platform gate and the schedule for this train is going to get off kilter.’ These predictions of impending disturbances are linked to another AI-enabled tool that does simulations that provide decision support for the network operations staff to assess such options as, ‘Do we just wait, and this problem will take care of itself? Do we need to inject another train onto this track to take care of any crowd build up on the platform? Do we need to bring in buses to shuttle stranded passengers on the platform to other MRT stations?’ The simulation model will evaluate and suggest these possibilities, but the network operators are the ones who make these decisions because they have greater awareness of the total situation and a more comprehensive and nuanced understanding of the implications of choosing one option over another under a given set of circumstances.”
He adds: “With this type of augmentation, the humans – the four people who monitor rail network operations – are still an integral part of the work process and very much in the evaluation and decision-making loop. With the support of this AI-enabled technology, this team of four people can now manage the entire rail network even though there are twice as many train stations as they had ten years ago when there were still four people in the rail network operations centre on each shift. That’s an example of augmenting and improving the productivity of operations.”
Professor Miller explained the concept of “so-so automation”, a term coined by two economists in Boston (Professor Daron Acemoglu at MIT and Professor Pascual Restrepo at Boston University) to describe the situation where an employer uses automation – include AI-enabled automation – to displace and likely retrench a worker to save some labour costs without attempting to use the automation to drive productivity improvement in ways that would result in increased requirements for existing work and the need to retain the worker.
He elaborates on this concept of so-so automation. “An employer might think, ‘I can get the tool in there and I can take out one headcount, or ten, or a hundred. And I stop there with some degree of labour cost savings. I don't want to bother to drive the technology deployment further to get a wider range of productivity improvements beyond some amount of labour cost savings. So I am not going to make the additional follow-on productivity enhancing efforts that would enable me to reduce price by five percent and get more customers, or enhance value by making my services better, again resulting in increased sales, and therefore create additional demand for existing work that enables me to retain my existing employees, even with the automation.
“And I don't want to put effort into using the automation to help me create new ways of doing things that results in new products or services and requirements for new types of work.” As a result, more technology usage would result in more automation and more displacement of existing work. This is why Professor Miller refers to this concept of “so-so” automation as a “lazy approach” to automation that in fact could lead to undesirable outcomes if enough employers had this limited mindset.
He emphasised, though, that based on his observations to date, it seems that more employers are using an augmentation approach rather than an automation approach in this current new wave of AI deployments. Professor Miller believes there are several reasons for this:
- While this new generation of machine-learning-driven AI systems extend the capabilities of what AI-enabled systems and machines can do, these methods and the statistics-based predictions and recommendations they produce are far from perfect and are therefore error-prone, and often require human checking and adjustment;
- Automation, even when it works well and even when enabled by this new generation of AI, still restricts the flexibility of a work process, and in many types of work, it still requires a human to provide the necessary adaptability, especially when operating conditions are constantly changing; and
- It is faster and easier to deploy augmentation than full automation because the human can compensate for the limitations of the AI-enabled machine whereas full automation requires an extremely high degree of accuracy, reliability and trustworthiness which can be quite expensive if not extremely difficult to attain.
Concerns with potential impacts on the wage distribution and nature of jobs
Professor Miller also pointed out that several labour economists and policy analysts who seriously study the impacts of technology on labour markets are of the opinion that the major impacts of AI will not be on the number of jobs per se, but rather on the nature and quality of jobs. In other words, the major labour market impacts are unlikely to be issues of mass job displacement and much more likely related to the way in which technology will change the profile of employment by occupation, and related to this, the resulting changes in the wage distribution both within and across occupations.
Recent studies of the US economy and other developed economies by labour market scholars have documented a “barbell effect” where there have been increases in the proportion of jobs in the highest paid occupational categories (which are also the highest skilled) and in the lowest paid occupational categories, with a decrease in the proportion (though not absolute number) of jobs in the middle wage categories. There is legitimate concern that this trend could continue.
In recent decades, again with reference to the US and other highly developed economies, there has been a reduction in the proportion of people employed who do the more routine types of clerical work and production work. Concurrently, there has been an increase in the proportion of jobs in various personal care, lifestyle and basic support services (home health care attendants, hospital aides, hairdressers, tattoo studio workers, fast food workers) and in gig jobs such as private hire drivers. While these types of service jobs create employment, Professor Miller notes: “They're not great jobs in terms of job quality. They usually don't have health benefits. They're low income.”
He commented that labour economists have shown that when people lose their jobs in the middle income-middle skill level occupational categories, they infrequently are able to get replacement jobs in the higher income occupational categories. Rather, they more often end up with a replacement job in the lower income categories which typically only require very general and basic human capabilities. “A lot of people in the middle have gotten squeezed. That's really the issue.” As these trends were documented even before the recent wave of machine-learning-driven AI has become widespread enough to have an economy-wide impact in any country, it raises the issues of what will happen going forward as these technologies become more widely used.
At the same time, in 11 of the 12 countries that currently comprise the world’s largest economies, the total fertility rate is below the replacement rate required to keep the population from shrinking and the proportion of people 65 and above is increasing relative to the younger segments of the population. This means that in many of the world’s largest economies, there is going to be a shortage of labour to keep the economy running even at current levels, not even considering growth. In fact, many of the highly developed countries already are experiencing a shortage of labour.
This is another reason why it is highly unlikely that increased usage of AI and all other forms of technology will result in mass job displacement as all types of human skill levels will be in demand, though not always at a desirable wage. And of course, there is a shortage of the highly skilled labour required to create new AI-enabled software tools and hardware machines for both automation and augmentation and to oversee their deployment. Those with these highly specialised and scarce in-demand skills will continue to draw increasing salaries while those doing more routinised middle skill work and lower income service jobs will likely continue to struggle to stay afloat, at least under current circumstances in the US and in a number of other countries.
Professor Miller is highly optimistic about the potential for using AI in the workplace to increase productivity and to support innovation. This in turn will very likely lead to an increase in many existing tasks and related jobs (which are a combination of tasks) via productivity-induced additional demand. It will also lead to the creation and increase in new types of tasks and corresponding new types of jobs via innovation-induced new work. He notes the recent analysis results of a team led by MIT labour economics professor David Autor that showed that recent employment levels in the US economy are more than three times higher than employment levels in 1940 (over 160 million vs 50 million) and that over 60 percent of the more recent levels of employment were in detailed occupational categories that did not exist in 1940 (e.g. semiconductor designer, computer programmer, solar panel installation specialist, wellness therapist). In other words, even with all the technology advances between 1940 and recently (as per 2018 employment and census data) which also included steadily increasing usage of increasingly capable types of automation, there were many new jobs created, and most of these jobs were created in new occupational categories that only came into existence after 1940.
The key point is that over the past 80 years and up to now, taking the US economy as the example, steadily increasing amounts of workplace technology and automation including vast amounts of computerisation and the use of the earlier generations of AI have co-occurred with increases in the overall number of people employed. Yet, the creation of opportunities for new types of work in many new types of occupations as a result of technology enablement and related innovation have led to new products and services and corresponding new work, and this has been a big part of the reason for this employment increase even with all of the increasing usage of technology for automation and augmentation. Also, other types of changes in consumer preferences and tastes, and changing demographic profiles, have also been a factor in creating some of these new types of jobs and occupations.
Yet, with this increase in overall employment and opportunities for new work, there has been a strong degree of skewing of the distribution of wages and total income across occupations with the highly paid occupations realising substantial relative and absolute gains and the middle income and lower income occupations substantially losing ground.
Professor Miller notes that these are the two big challenges that every society will face as they intensify and accelerate their usage of AI-enabled technology in the workplace. First, can they create a sufficient amount of new work – new jobs in new occupations – to more than offset the substantial subset of existing types of jobs that are more likely to be fully automated? Or if not fully automated, be so highly augmented that labour requirements for that occupation decline nonetheless. Secondly, can they do this without substantially increasing an already highly skewed distribution of wages across occupational categories? Can this be done in ways that lead to growing opportunities for the middle and lower ends of the wage spectrum so that there is a lower degree of economic inequality across the population?
He points out that in addressing these two challenges, there are advantages to highlighting and promoting the opportunities for using AI-enabled technology for augmentation rather than full automation. An augmentation approach helps to retain jobs. It helps to make those jobs more valuable because the people who do them can produce more in terms of both quality and quantity. And the combination of adaptable and context-aware people working together with AI-enabled tools is well suited for doing the type of workplace collaboration, exploration and experimentation required to innovate and to adapt to changing circumstances.
He notes: “The extent to which we drive automation versus augmentation in an important factor in how we go forward. It all comes down to choices. Employers need to realise that full automation is not the only alternative, and that while it has its place and appropriate usage, in many circumstances and settings, it is not the most effective alternative vis-à-vis well-designed augmentation. These choices of automation versus augmentation and resulting outcomes are not pre-determined by the technology per say.
“They depend on how people and institutions choose to make use of the technology. Every employer makes their own choices about how they will use AI in combination with other workplace technologies. Also, governments make choices about policies which will influence the extent to which there are stronger incentives for automation and replacing labour with capital versus for augmentation and amplifying the capabilities and value of existing workers.”
He surmises: “There's no way to avoid using this new wave of AI in combination with all other types of existing and new technology. We're going to end up using it more and more for a whole host of reasons including the need to drive productivity increase, the need for innovation to create new products and services, and the need to address and respond to pressing problems that threaten the survival of companies as well as the survival of the human race. Our challenge is to go about this in a way that creates opportunity and livelihoods more broadly for all segments of a country’s population so that the social fabric both within and across countries is not further unravelled through increasing degrees of inaccessibility of opportunity and resulting inequality.”
Back to Research@SMU November 2022 Issue
See More News
Want to see more of SMU Research?
Sign up for Research@SMU e-newslettter to know more about our research and research-related events!
If you would like to remove yourself from all our mailing list, please visit https://eservices.smu.edu.sg/internet/DNC/Default.aspx