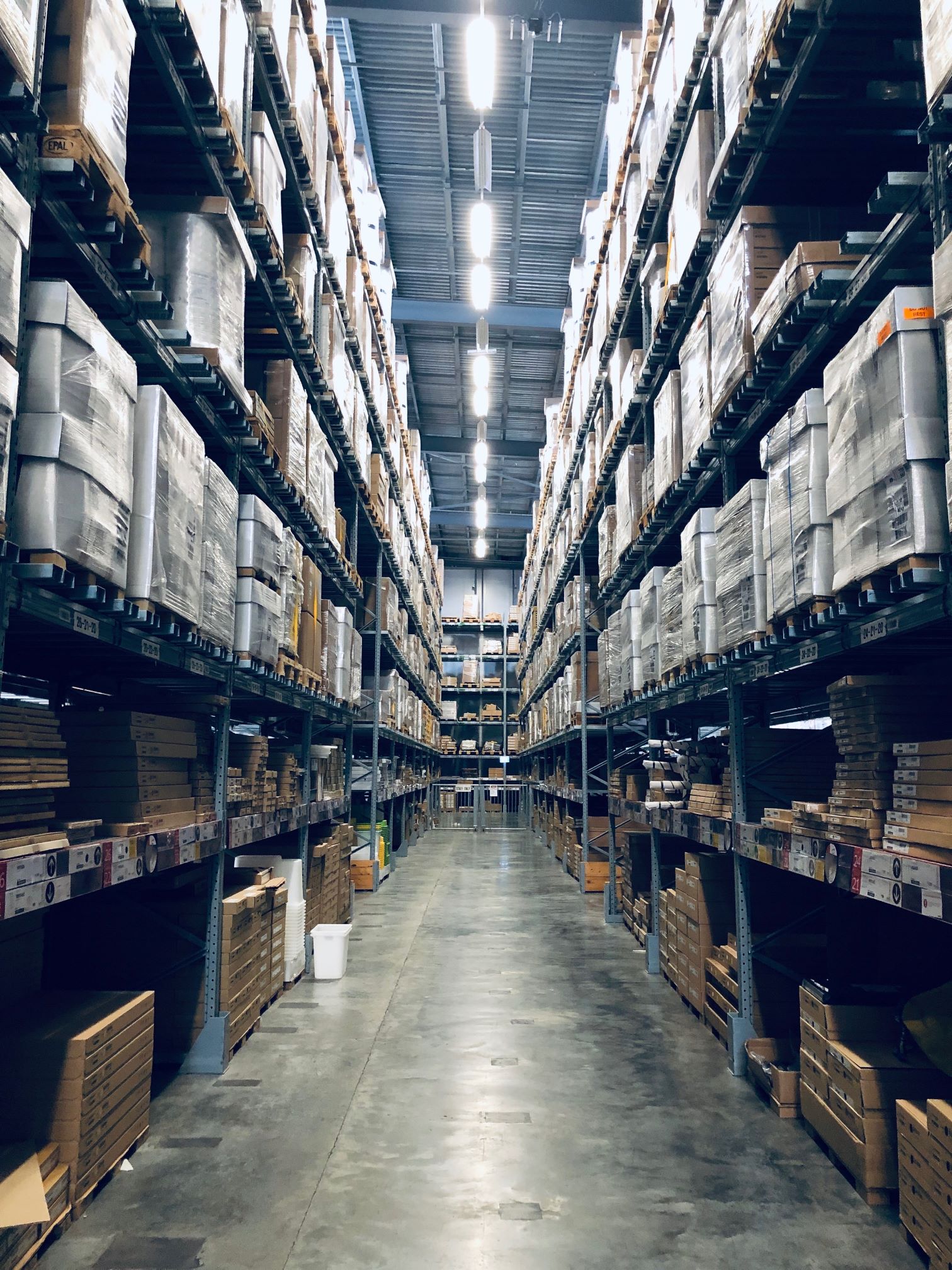
With the rapid growth of online shopping, e-commerce retailers face shifting operating costs and challenges, including shipping across geographic regions and time periods.
Research conducted by Yun Fong Lim and Marcus Ang from Lee Kong Chian School of Business, Singapore Management University and Song Jiu from the School of Economics and Management, Southwest Jiaotong University explores strategies to improve how online stores manage their inventory and orderst.
Their research concept aims to improve the efficacy of online retailing operations by predicting future demand for products and replenishing inventory to prevent shortages, while also quickly fulfilling orders as they come in.
Read their original research: https://doi.org/10.1287/msom.2020.0926
Transcript:
Hello and welcome to Research Pod. Thank you for listening and joining us today.
In this episode, we explore strategies to improve how online stores manage their inventory and orders through the research conducted by Yun Fong Lim and Marcus Ang from Lee Kong Chian School of Business, Singapore Management University and Song Jiu from the School of Economics and Management, Southwest Jiaotong University. Their research is related to Integrating Anticipative Replenishment Allocation with Reactive Fulfilment for Online Retailing – using a research concept that aims to improve the efficacy of online retailing operations. The concept involves combining anticipative replenishment-allocation with reactive fulfilment strategies using robust optimisation techniques.
Robust optimisation is a methodology used to improve how online stores manage their inventory and orders. It involves predicting future demand for products and replenishing inventory to prevent shortages, while also quickly fulfilling orders as they come in. The approach uses mathematical techniques to make these processes more efficient and reliable.
Due to the rapid development of the internet and technology, including mobile phones, more and more consumers prefer to shop online. The exponential growth in sales makes e-commerce an attractive industry. However, this growth also entails a significant increase in operating costs. The research developed by the team is motivated by the challenges of online retailers who sell their products in such a way that demand is met in different geographical zones over multiple time periods. But, what is exactly the issue and why do the online retailers’ operating costs increase? Well, the retailer replenishes the products from different suppliers and stores them in several fulfilment centres to meet the demand.
In each period, the retailer is confronted with three types of decisions. At the beginning of a given period, the retailer determines the quantity to replenish for each product from each supplier, considering the lead time and the limitations on production capacity. In the next step, the retailer will decide the quantities of stock to distribute to the various fulfilment centres, always considering the capacities of the fulfilment centres and allocation costs. At the end of the period, the demand is known and the retailer, after analysis, makes its decision on which fulfilment centre will satisfy the demand. If a product is out of stock, the retailer requests the product to be drop-shipped from suppliers to satisfy the demand.
The researchers approached this problem not only in a theoretical way but also through interaction with their industrial partner, which is a major fashion online retailer in Asia. The retailer serves the demands of six countries through three fulfilment centres strategically located in different Asian cities. As one would expect, the retailers' objective is to minimise the expected total operating cost over the horizon of multiple periods.
With this goal in mind, there is a challenging problem to be solved. Without knowing the demand for its products, in each period an online retailer has a tough task of deciding how much to replenish for each product and what is the most efficient way to allocate the inventory across the fulfilment centres. And, after the demand in a given period is known, the retailer decides on which fulfilment centres should be used.
Online retailers face a complex puzzle to minimise their total operating cost, which requires orchestrated optimisation of replenishment, allocation, and fulfilment decisions. A big part of the problem is that replenishment and allocation are done in an anticipative manner under a “push” strategy. However, the fulfilment is executed in a reactive way under a “pull” strategy.
To solve this problem, the authors propose a multi-period stochastic optimisation model. This is a mathematical model that is used to make decisions over multiple time periods in the presence of uncertainty. The "stochastic" aspect incorporates random variables to account for uncertain future events. The "optimisation" aspect aims to find the best possible decisions or strategy, based on some objective function or criteria. Overall, a multi-period stochastic optimisation model is a powerful tool for decision-making in complex, uncertain environments, and is widely used in areas such as finance, operations management, and engineering.
Lim, Ang, and Jiu are the first to establish a multi-period stochastic optimisation model that integrates the anticipative replenishment-allocation decisions with the reactive fulfilment decisions. In online retailing, it is common to restock and distribute products before the actual demand is known, and then fulfil orders after the demand is clear. This research fills a major gap in the online retailing literature in which all studies address the replenishment, allocation, and fulfilment problems separately.
The researchers suggest an automatic way to make decisions about restocking products and fulfilling orders as demand changes over time. They use an optimisation model to make these decisions seamlessly. Moreover, the fulfilment flexibility adds even more complexity to the inventory allocation to the fulfilment centres and the product replenishments from the suppliers.
Unlike brick-and-mortar retailing, where business owners showcase their products using a physical storefront, a distinctive characteristic of online retailing is that the retailer can be flexible to satisfy the demands of a zone from any warehouse that holds the inventory at a given time. This fulfilment flexibility improves service levels, but may also increase the retailer’s shipping cost, which is a main operating cost in this industry.
The researchers propose a solution to this problem based on three dimensions. First, the stochastic optimisation model, which helps the retailer to minimise the total operating cost when facing randomness, is developed. Second, an efficient and scalable solution approach is proposed. Finally, technical insights are obtained.
Robust optimisation is a methodology used to deal with optimisation problems when uncertainty is present. It uses limited information about uncertainty to develop manageable models. In addition, this approach makes it possible to meet specific predetermined objectives.
In their study, the researchers propose a two-phase approach based on robust optimisation to solve the joint replenishment, allocation, and fulfilment problem for online retailing. In the first phase, the approach is to use a static rule which is a set of predetermined decisions or unchangeable instructions. The static rule is a fixed rule that is applied uniformly regardless of any external factors or circumstances. This phase decides whether the products should be replenished in each period. In the second phase, the proposed approach uses a linear decision rule to determine continuous decisions. In other words, the decision-making process is based on a linear function that maps input variables to an output decision. In this phase, binary decisions are fixed when determining the replenishment, allocation, and fulfilment quantities.
The numerical experiments showed that the proposed model is better than other methods found in previous studies in terms of the online retailer's operating cost and the computational speed. A case study using real data from a major fashion online retailer in Asia suggests that the two-phase approach can potentially reduce the retailer’s cumulative cost significantly.
The team’s research is unique in a way that it decouples binary decisions from continuous decisions. By decoupling binary from continuous decisions, the proposed methodology can solve large problems with up to 1,200 products, resulting in substantial cost savings for online retailers. This approach has demonstrated promising performance in producing high-quality solutions while preserving tractability, and helps boost the online retailer’s competency in this cut-throat industry. Additionally, the integration, robustness, and adaptability of the decisions under the approach is of significant value.
Lim, Ang, and Jiu´s innovative research proposes that we can improve how online stores manage inventory and orders by using mathematical techniques to better predict future product demand, replenish inventory, and quickly fulfil orders as they come in. The team uses a multi-period stochastic optimisation model to integrate anticipative replenishment-allocation decisions with reactive fulfilment decisions. Moreover, they propose a unique solution based on a robust optimisation methodology to deal with the uncertainty of demand. Online retailers will benefit from this approach by increasing efficiency and minimising costs, while improving service levels, meaning they may become increasingly more competent in the future.
That’s all for this episode, thanks for listening. Don’t forget to check out the links to the original research in the show notes for this episode, and stay subscribed to ResearchPod for more the latest science.
See you again soon.
Also published on: https://researchpod.org/informatics-technology/anticipative-replenishment-reactive-fulfilment
Podcast is also available on Spotify, Apple iTunes, Google Podcasts, and many more (please use search term “ResearchPod”).
See More News
Want to see more of SMU Research?
Sign up for Research@SMU e-newslettter to know more about our research and research-related events!
If you would like to remove yourself from all our mailing list, please visit https://eservices.smu.edu.sg/internet/DNC/Default.aspx