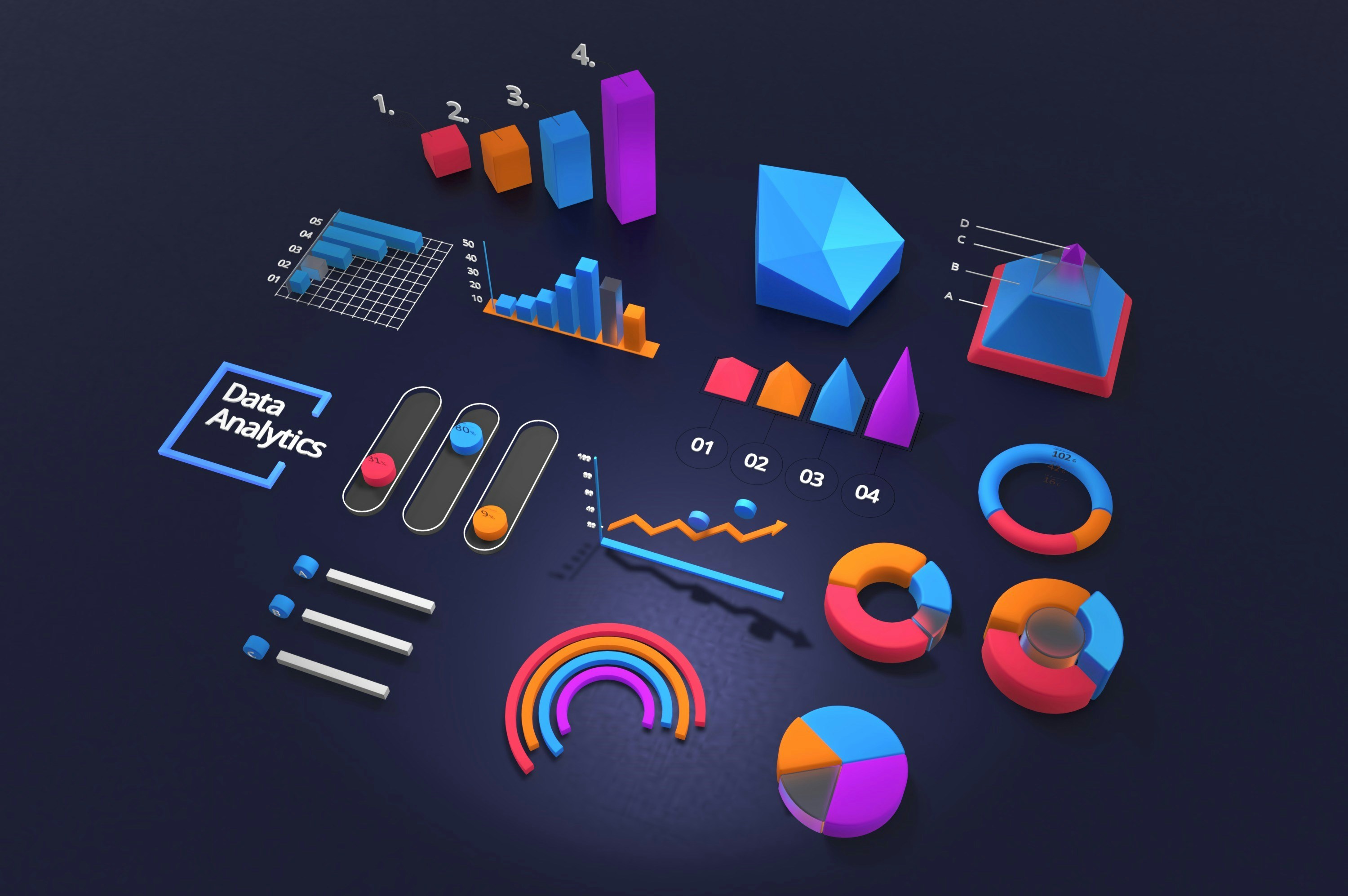
‘Big Data’ has appeared more and more throughout boardrooms and executive suites, in order to determine how organisations extract value from information. Its potential impact on a firms’ earnings forecasts is of great discussion, but no direct link to forecast frequency has been made yet.
Associate Professor Goh Beng Wee from Singapore Management University examines the interplay between firms’ engagement in data analytics and their voluntary disclosure behaviour.
Read the original article: doi.org/10.2308/HORIZONS-2020-145
Transcript
Hello and welcome to ResearchPod. Thank you for listening and joining us today.
In this episode, we look at a thought-provoking study by Associate Professor Goh Beng Wee, an experienced academic from the Singapore Management University. Over recent years, the topic of “Big Data” and “data analytics” has appeared throughout boardrooms and executive suites, in order to determine how organisations extract value from information. Today, we delve into the world of Big Data analytics and its impact on contemporary business landscapes.
Defined as the complex process of dissecting datasets to show hidden patterns, correlations, and market trends, Big Data analytics produces a new era of informed decision-making. Empowered by the convergence of expansive data and analytical tools, businesses increasingly integrate these insights into their operational frameworks.
As underscored by Chen, Chiang, and Storey in 2012, and corroborated by the 2018 McKinsey Global Survey on data and analytics, the utilisation of Big Data analytics isn’t just a trend, but a strategic move for a business. It catalyses growth, transforms business practices, and reshapes competitive dynamics. Dr Goh’s work examines the interplay between firms’ engagement in data analytics and their voluntary disclosure behaviour.
In light of the interest in leveraging Big Data analytics for strategic advantage, its potential impact on a firms’ propensity to issue management earnings forecasts is of great discussion. The direct linkage to forecasting earnings or improving voluntary disclosures remains inconclusive. However, the team from Singapore Management University suggest that Big Data analytics may influence the issuance of management forecasts through two distinct yet interconnected channels.
First, the “supply channel” argument suggests that, although forecasting earnings may not be a primary objective of Big Data initiatives, the spill over effects of comprehensive data analytics could enhance firms’ forecasting capabilities. By integrating predictive analytics into decision-making processes, organisations can anticipate market dynamics and operational outcomes with greater precision. This mitigates the fear of inaccurate predictions and reduces the costs associated with disclosure.
Second, the “demand channel” argument shows that understanding the impact of Big Data analytics on financial outcomes could drive firms to provide more transparent disclosures, including management earnings forecasts. However, factors, such as the speed of information transmission, increased uncertainty from statistical correlations, and proprietary concerns, may impede the association between Big Data analytics and management forecasting.
Therefore, in order to understand the relationship between Big Data analytics usage and the ability to issue management earnings forecasts, Dr Goh formulates the following null hypothesis: There is no association between Big Data analytics usage and the propensity to issue management earnings forecasts.
In the world of corporate disclosures, an observable trend emerges in the form of qualitative discussions within annual reports, which sheds light on the strategic adoption of data analytics. Leveraging insights from the extensive literature and annual reports, the study seeks to understand Big Data utilisation, drawing from methodologies refined by scholars such as Li, Hoberg, and Bushman.
Companies have comprehensive annual reports that detail their financial performance. This is called a 10-K. Dr Goh uses Analytics_Indicator and Analytics_RankWords as measures for examining Big Data Analytics. But what are they? Well, Analytics_Indicator is defined as “an Indicator variable that equals 1 if the firm disclosed any of the key words related to Big Data analytics in its 10-K for a given year, and 0 otherwise” and the Analytics_RankWords is defined as “Quartile ranking of the ratio of key words on Big Data analytics to the total number of words in the 10-K”.
However, there are limitations of the measures. They may not fully capture the intricacies of Big Data adoption. Despite these challenges, Dr Goh’s approach enables researchers to understand the ever-changing landscape of corporate data strategies, and insights into the topics of technology, strategy, and disclosure practices.
In this latest analysis, a probit regression model is utilised to assess the relationship between firms’ engagement with Big Data analytics and their ability to issue management earnings forecasts. The regression equation examines the likelihood of firms issuing such forecasts after discussions on Big Data analytics in their 10-K filings. Within the study, the author aims to discern whether firms actively involved in data analytics demonstrate a heightened propensity for issuing earnings forecasts. Additionally, this research incorporates controls for various determinants of forecast issuance, along with industry and year fixed effects. The SMU team approach shows their commitment to explore the dynamics of corporate disclosure practices in Big Data analytics.
One of the study’s main results shows that the relationship between firms’ utilisation of Big Data analytics and their ability to issue management earnings forecasts. The Analytics_Indicator signified a positive and statistically significant association, indicating that firms disclosing Big Data analytics discussions are more inclined to issue earnings forecasts. Similarly, Analytics_RankWords remains positive and significant affirming the hypothesis. The economic significance of this is that firms that used Big Data Analytics has a 4% increased likelihood of issuing forecasts over those that didn’t.
The next topic to look at is the “supply channel” argument. Let’s delve deeper into the impact of Big Data analytics on management forecast accuracy. By assessing the regression model with management forecast error (MFE) as the dependent variable, Dr Goh’s analysis sheds light on the relationship between data analytics and forecast precision. Findings corroborated the supply channel hypothesis by showing that Big Data analytics does indeed enhance the accuracy of management earnings forecasts.
The study shows the significance of the role played by financial intermediaries, particularly financial analysts, in fulfilling investors’ information demands. Therefore, if investors exhibit an increased interest in firms engaged in Big Data analytics, one can anticipate a corresponding increase in analyst following. Dr Goh’s results show that both Analytics_Indicator and Analytics_RankWords exhibit positive coefficients, indicating a higher analyst following for firms immersed in Big Data analytics. These findings demonstrate the multifaceted impact of data analytics on information dissemination, again, showing the link between investor demand and firm disclosures. Ultimately, Dr Goh’s analysis shows a dual relationship between disclosure costs and heightened investor demand. This shows a positive association between Big Data analytics and management forecasting.
In the quest for deeper insights, Dr Goh delves into additional analyses to uncover the factors that moderate the relationship between Big Data analytics and management forecasting. Findings reveal that the relationship is greater in larger, more complex, and well-governed firms, when compared against their smaller, counterparts. Furthermore, the investigation extends to the sales forecasts, uncovering a similar association with the adoption of Big Data analytics.
On the path of Big Data, the study showcases the benefits accrued to companies, that encompass operational efficiency, adopt swifter decision-making, create quality customer experiences, and increase revenue streams. Importantly, Dr Goh highlights the often-overlooked advantage of improved communication with capital markets, a topic that warrants greater consideration in Big Data investment decisions. Encouraging business leaders to adopt a holistic perspective, Dr Goh’s findings show the potential benefits of Big Data utilisation. Nonetheless, the author acknowledges the limitations of the study and advocating for future research especially in regard to the balance required between costs and benefits in Big Data investments.
That’s all for this episode, thanks for listening. Links to the original research can be found in the show notes for this episode. Stay subscribed to ResearchPod for more of the latest science.
See you again soon!
Also published on: https://researchpod.org/business/big-data-analytics-management-forecasting-behaviour
Podcast is also available on Spotify, Apple iTunes, Google Podcasts, and many more (please use search term “ResearchPod”).
See More News
Want to see more of SMU Research?
Sign up for Research@SMU e-newslettter to know more about our research and research-related events!
If you would like to remove yourself from all our mailing list, please visit https://eservices.smu.edu.sg/internet/DNC/Default.aspx