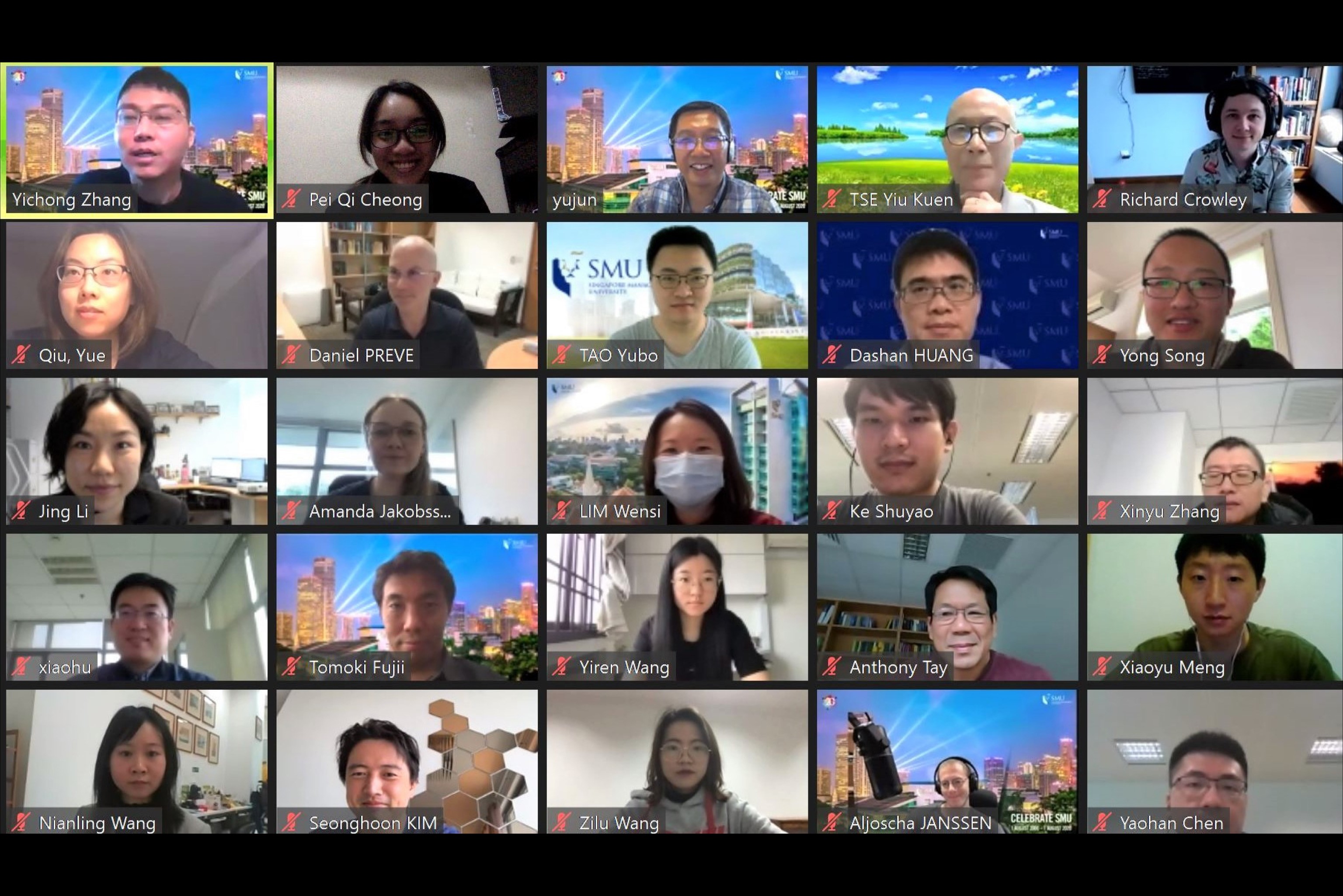
By Sheryl Lee
SMU Office of Research & Tech Transfer – Even as the first batch of COVID-19 vaccines arrived in Singapore last December, reports of a mutant strain from the UK were already surfacing. Such is the unpredictability of an evolving pandemic, which has permeated every aspect of our lives. Even our economic systems have not been spared, with the uncertainty manifesting itself in massively divergent economic forecasts. Currently, the forecast spread among analysts is far larger than at any point during the past 20 years, including the 2008 financial crisis.
With such a diverse range of predictions, how should central banks like the Monetary Authority of Singapore (MAS) condense forecasts into a single summary value for decision makers? One way is to simply pick the median value, but many other methods abound in this field of study known as forecast combinations. Approaches range from calculating the average to assigning different weights, and even include machine learning techniques, as SMU Professor Jun Yu demonstrated in the School of Economics’ inaugural virtual public lecture on 8 December 2020.
Solving the forecast combination puzzle
When multiple forecasts are summarised into one combined figure, we enjoy reduced complexity. But some information is lost during this process – whether the data was skewed; how variables relate to each other; whether forecasts from certain models outperform others.
To minimise information loss, researchers have tried estimating and assigning individual weights to each forecast. However, empirical studies have shown that these do not always perform better than the simple average, or equally weighted approach. This phenomenon is known as the forecast combination puzzle.
To unlock this puzzle and beat the simple average, Professor Yu and his collaborators have proposed a novel approach that uses forecast combination techniques to combine the outputs of multiple machine learning methods. Professor Yu says he chose machine learning models such as regression trees and support vector machines over conventional econometric ones because they are more data-driven, relying on patterns to make inferences instead of imposing a rigid set of assumptions on the data.
“Machine learning is an algorithmic approach to perform specific tasks without using explicit instructions. The specific task here in our context is economic forecasting,” he explains. In economic and other types of data where relationships between response and explanatory variables are too complex to be captured by simple linear models, machine learning techniques can be “very powerful,” he says.
An all-in-one algorithm
While machine learning techniques have found success in economic forecasting in recent years, they too have their limitations, Professor Yu says. For example, most machine learning methods assume stability in underlying relationships between variables. This may not hold true because the economy is usually in flux, affected by business cycles and policy interventions.
“It is possible that the best machine learning strategy in one period may not be the best in another period,” Professor Yu concludes. Such model uncertainty makes choosing any one machine learning model too risky, and this is also where forecast combination techniques come in: by generating predictions from multiple models and summarising them into one combined forecast, we can not only reduce complexity, but also increase the reliability of our economic predictions. In a working paper titled Forecast combinations in machine learning, Professor Yu and team introduced a new ensemble learning algorithm that combines outputs from various machine learning methods to achieve one summary forecast figure.
Applying their novel framework to both simulation and empirical studies, Professor Yu and his collaborators found that their proposed approach produced more accurate forecasts than both individual machine learning methods and the simple averaging technique – effectively unlocking the forecast combination puzzle for macroeconomic predictions.
Half science and half art
As acclaimed British statistician George Box once declared, all models are wrong, but some are useful. Quoting Box in his lecture, Professor Yu adds that this is especially true for econometric models, which are complex, wide-ranging and involve many economic agents. “This fact makes building a good econometrics model half science and half art,” he shares.
Using a blend of machine learning methods and forecast combination techniques, Professor Yu and his collaborators have improved on both the science and art of economic modelling. Walking the audience through some empirical studies, he explains how the team first obtained a list of multiple expert forecasts from MAS detailing one-quarter-ahead predictions of Singapore’s real gross domestic product growth, then derived a combined forecast from multiple machine learning methods using this data.
Comparing their results with the median value of the forecasts, traditionally the summary value provided by MAS, Professor Yu found the team’s predictions to be more accurate, with figures closer to the actual reported values. “We are doing much better than the median of professional forecasters,” he enthuses. “Applying forecast combination techniques to machine learning strategies helps us to improve economic forecasts.” Similar gains in predictions have been found in forecast other macroeconomic variables and financial variables.
Back to Research@SMU Feb 2021 Issue
See More News
Want to see more of SMU Research?
Sign up for Research@SMU e-newslettter to know more about our research and research-related events!
If you would like to remove yourself from all our mailing list, please visit https://eservices.smu.edu.sg/internet/DNC/Default.aspx