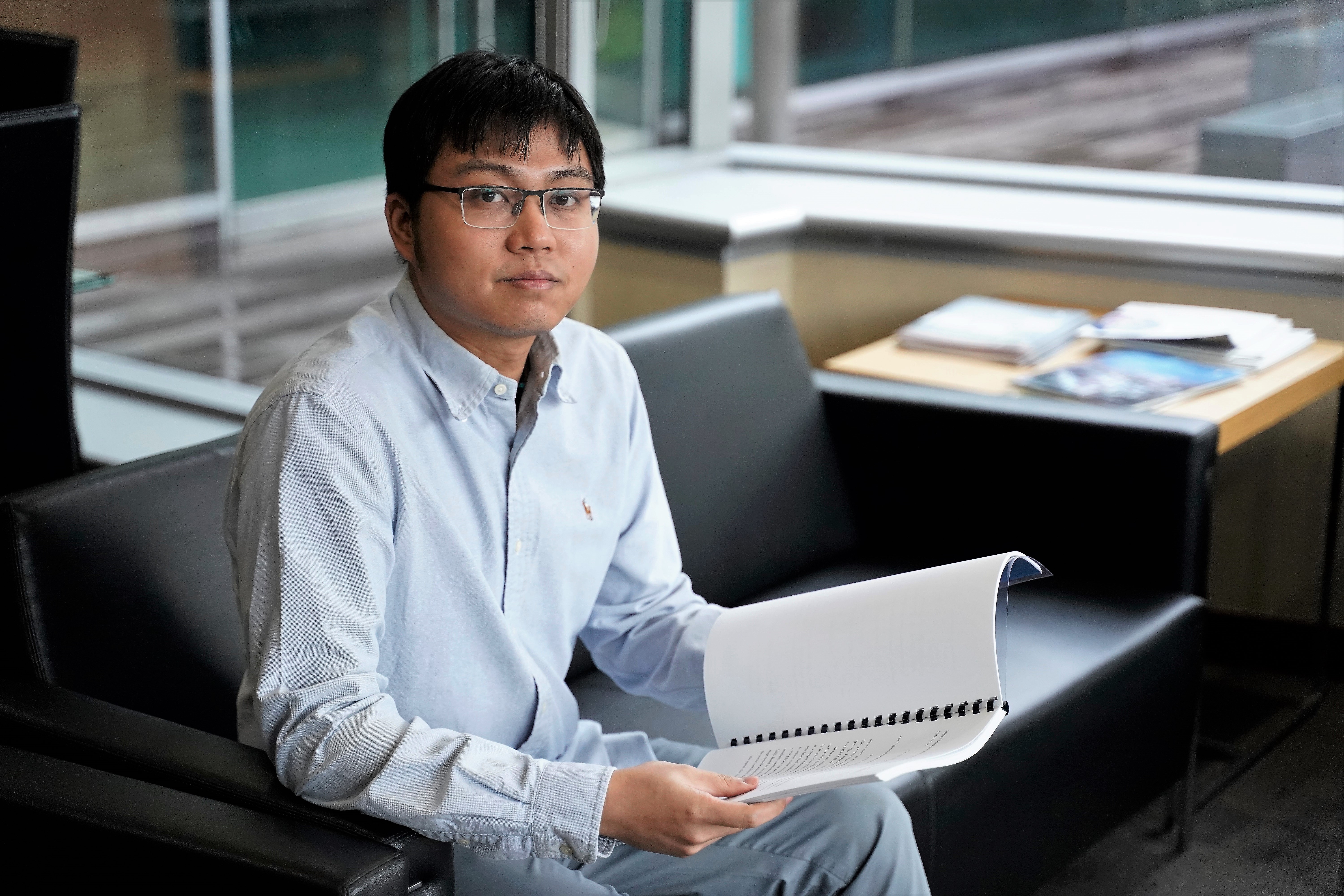
By Sim Shuzhen
SMU Office of Research & Tech Transfer – In many countries, education levels and wages are linked – economists might expect people with a university education to earn more than those who did not complete high school, for example. But policy makers are often interested in the finer details – how much bigger does your pay cheque get with each additional year of education? What is the wage gap between graduates and non-graduates by the time they reach middle age?
To glean insights at this level, researchers turn to econometrics, a discipline which combines economic theory, statistical inference methods and mathematics to quantify economic relationships.
“Research in econometrics emphasises both rigorous mathematical derivations and meaningful applications,” says Assistant Professor Zhang Yichong, an econometrician at the Singapore Management University (SMU) School of Economics. “The former ensures that the research is falsifiable [i.e. testable], and the latter ensures that the research has practical value.”
For Professor Zhang, the appeal of econometrics is that it straddles both theory and practice. “I was trained to appreciate the pure beauty of logic and reasoning,” he explains. “However, I personally also value practicality and am seeking meaning in my work. Therefore, it is obvious for me to choose econometrics as my subject of interest.”
When classical methods fail
Professor Zhang specialises in developing new statistical methods for econometrics, which researchers can turn to as theoretically valid, practically reliable alternatives in situations when classical statistical methods fail.
For example, classical methods to estimate treatment effects stumble when the data in question come from the ‘tails’ or extreme quantiles of the distribution – public health researchers may be interested in infants with low birth weights, and education policy makers in students with low test scores, for instance. “In this case, researchers encounter not only the usual missing counterfactual [a problem where the outcome under an alternative treatment is unknown] but also data sparsity, because there are not many observations in the tails,” says Professor Zhang.
In a study titled ‘Extremal Quantile Treatment Effects’, published in 2018 in The Annals of Statistics, Professor Zhang established a new method to estimate tail quantile treatment effects at the lowest ranks of a distribution. The method, based on asymptotic theory and inference, resolves both the missing counterfactual and the data sparsity problems.
“I addressed both issues by studying the limiting behaviour of the quantile treatment effect estimator under a drifting sequence framework, which can approximate reality better than the classical framework,” explains Professor Zhang.
Testing at the tails
For econometric methods to be practically useful, researchers need to be able to apply them to real-world data to test hypotheses. To evaluate his new method of estimating quantile treatment effects, Professor Zhang first applied it to birth data to examine the effect of maternal smoking on the lower tail of babies’ birth weights.
While maternal smoking has often been linked to low birth weights—a major cause of infant mortality – there is no consensus as to the magnitude of this effect, explains Professor Zhang. Applying the new method, he found that maternal smoking has no statistically significant effect on babies’ lowest possible birth weights.
Next, Professor Zhang used the method to examine how California’s Proposition 209 – which in 1996 effectively banned the use of race as a factor in college admissions – affected the extent of affirmative action at University of California (UC) campuses. To study this, he focused on minority students with the lowest college preparation scores, who would be most affected by the new legislation.
Before Proposition 209, there is significant evidence that the UC campuses practiced affirmative action, implementing racial preference during college admissions decisions, Professor Zhang found. After voters passed the legislation, however, a more complex picture emerged.
“For higher-ranked campuses such as Berkeley and Los Angeles, the racial gaps [in college preparation scores] did not decrease, while for the middle-ranked campuses such as San Diego, the decrease was significant,” says Professor Zhang; this suggests that the individual UC campuses differed in terms of how they modified their admissions criteria post-Proposition 209.
Versatile and varied
That Professor Zhang’s new quantile treatment effect estimator can be applied to such diverse problems indicates how versatile econometric approaches can be. In addition, econometrics is also a fast-evolving discipline, such that Professor Zhang’s biggest challenge lies in keeping up with the latest developments, he says.
“Econometrics is an interdisciplinary field of economics and statistics. In the new era of big data and machine learning, many new inference methods, algorithms, and ideas are invented. In order to incorporate these methods into economic research, I need to be ready to acquire new knowledge at any time.”
One of Professor Zhang’s current projects involves estimating quantile treatment effects in covariate-adaptive randomised control trials, where individuals (patients in clinical trials, for example) are first stratified according to certain baseline criteria, such as stage of disease, and then assigned a treatment status.
“To achieve balance [within each stratum], individuals are not independently assigned, which causes cross-sectional dependence of the data, so that the classical hypothesis testing procedure becomes conservative,” he explains. “I am working with my student at SMU on developing a theoretically valid and practically easy-to-implement inference method in this scenario.”
Zooming out from the math to the bigger picture, it’s clear that econometric approaches can be very powerful, using data to estimate economic models, test economic theories, predict potential economic outcomes and make relevant policy recommendations, says Professor Zhang. “Econometrics has become the basic toolkit for most applied economists. With the influx of data in the big data era, it can be expected that econometrics will play a more and more critical role in the development of economic research.”
Back to Research@SMU February 2019 Issue
See More News
Want to see more of SMU Research?
Sign up for Research@SMU e-newslettter to know more about our research and research-related events!
If you would like to remove yourself from all our mailing list, please visit https://eservices.smu.edu.sg/internet/DNC/Default.aspx