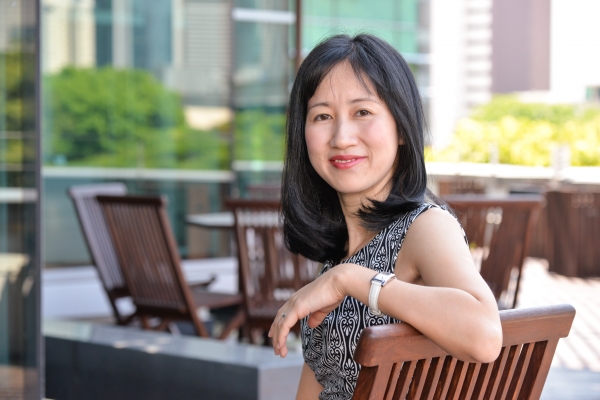
By Alistair Jones
SMU Office of Research – The terminology of artificial intelligence (AI) and its many acronyms can be confusing for a lay person, particularly as AI develops in sophistication.
Among the developments is deep learning – a machine learning technique that teaches computers to learn by example.
“Deep learning has brought many major changes to AI, especially in natural language processing (NLP) and computer vision, two sub areas of AI,” says Jing Jiang, a Professor of Computer Science at Singapore Management University (SMU).
“In my field, which is NLP, the solution approaches to many tasks have fundamentally changed due to the recent success of ChatGPT type of technologies, and deep learning is one of the key enabling factors to these technologies.”
ChatGPT is a prominent AI-powered chatbot that can generate human-like responses to text inputs in a conversational manner. Its outputs can include articles, reports and even song lyrics, though its attempt to 'write' a Nick Cave song was met with derision from the artist.
But ChatGPT continues to be improved. It draws its knowledge from massive data sets known as large-scale pre-trained language models (LLM) that tech corporations and governments have been building. It repurposes the data using generative AI, which produces the aforementioned articles and reports.
“ChatGPT was not intentionally trained to perform all these tasks. Its ability to transfer its knowledge learned from other tasks to a new task is an example of zero-shot transfer,” Professor Jiang says.
ChatGPT and its ilk have paved the way for a new research project led by Professor Jiang, which was recently awarded a MOE Academic Research Fund Tier 2 grant.
Professor Jiang is focusing on vision question answering (VisualQA), a technology that enables machines to answer questions based on visual data. The project aims to develop a new methodological framework to harness the power of large-scale pre-trained vision-language models (PT-VLM).
Discovering skillsets
Will this project be another case of generative AI?
“For certain types of questions, the answers do not need to be generated but are rather selected from a set of candidate answers,” Professor Jiang says.
“For example, if a question is asking about the colour of the followers in a picture, the answers can be chosen from a set of known colours.
“On the other hand, there are also some questions, especially those 'why' and 'how' questions, which may need answer generation because the answers to these questions are long sentences that cannot be directly chosen from any set of known answers. Therefore, in my project I will explore the use of existing generative language models for answer generation.”
The research team will identify the basic skills required by VisualQA and use a 'probing approach' to discover the 'skillsets' within the various pre-trained vision-language models. They will then design methods based on adapter modules, which are additional lightweight neural network layers, to augment pre-trained models with additional skills.
Necessary skills would include object recognition and spatial reasoning. Another more elusive skill is ‘common sense’. Can an algorithm replicate how humans think and behave in a reasonable way?
“This may sound like a daunting task, but researchers have been looking into this direction for quite a while,” Professor Jiang says.
“There are already a few resources available that try to capture commonsense knowledge, such as ConceptNet. Increasingly, people also find that LLMs themselves can capture commonsense knowledge, which they probably learned from the tremendous amount of data they are trained on.”
Commercial interest
Research and investment in PT-VLMs have lagged behind language models. Professor Jiang sees a number of reasons.
“First of all, language data is much more dense than visual data in terms of the amount of information or knowledge captured. This means that when trained on the same size of data, a language model could learn more human knowledge from the data than a vision model,” she says.
“Second, most human knowledge is still captured in textual format rather than in visual format, giving much more available training data to language models than to vision models or vision-language models.
“Third, verbal communication (including typing) is probably the most convenient and efficient way for humans to interact with machines, which means industry players will also focus more on developing powerful language models as foundations for their end-user products such as search engines and chatbots.”
Commercial interest in vision-language models has been growing because of the many potential use cases.
“One example is multimodal chatbots, which can receive inputs from humans not only in the format of speech and text but also in visual representations such as images and videos. Microsoft’s new Bing is a multimodal chatbot,” Professor Jiang says.
“Another important use case is embodied AI, where AI models sit on robots that can move around to sense their surroundings and perform tasks for humans. Joint vision-language AI models would enable an embodied AI agent (the robot) to understand a human’s verbal requests in the context of its surroundings.”
Practical impacts
Existing large-scale PT-VLMs are still not powerful enough on their own to handle many VisualQA questions and provide correct or relevant answers.
“The approach we see that is promising currently is to combine the powers of different pre-trained models, such as a framework called Visual ChatGPT developed by Microsoft,” Professor Jiang says. “The Visual ChatGPT framework does not attempt to further enhance the abilities of a single AI model. Rather, it leverages the different abilities of different pre-trained AI models to jointly perform a task such as modifying an interior design image based on a user’s verbal requests (e.g., “Replace the glass side table beside the sofa chair with a wooden one of similar size”).
“Here we can use one AI model for visual object detection, another for spatial reasoning, and a third for image generation, for example. The challenge lies in the dynamic decomposition of the original complex task into several simpler subtasks and the selection of suitable pre-trained AI models for each subtask. Visual ChatGPT uses ChatGPT model to perform the task decomposition and model selection, which I think is very smart.”
And then there is the issue of where to source new training data to augment PL-VLM models.
“It could be through repurposing existing datasets or through annotating new datasets by crowdsourcing. Because the field is evolving very rapidly, we will need to stay agile and be open to new ideas,” Professor Jiang says.
A well-known thorny issue is social biases within data sets.
“It is not easy to mitigate these biases. The problem is also complicated [because] social biases are different in different societies and cultures. Nevertheless, companies developing large pre-trained models are proactively removing or reducing these biases through human interventions.”
Professor Jiang envisages practical impacts from her research project.
“I believe the research output from my project can be used to improve multimodal chatbots and embodied AI agents. These bots can be particularly useful for increasing productivity in sectors such as retail, hospitality, education and healthcare,” she says.
“I currently have another ongoing project that aims to build a virtual avatar to interact with people living with dementia. VisualQA technologies are an important component of such virtual avatars. For societies such as Singapore that are facing imminent ageing problems, these AI-powered social bots have many potential applications.”
Back to Research@SMU May 2023 Issue
See More News
Want to see more of SMU Research?
Sign up for Research@SMU e-newslettter to know more about our research and research-related events!
If you would like to remove yourself from all our mailing list, please visit https://eservices.smu.edu.sg/internet/DNC/Default.aspx