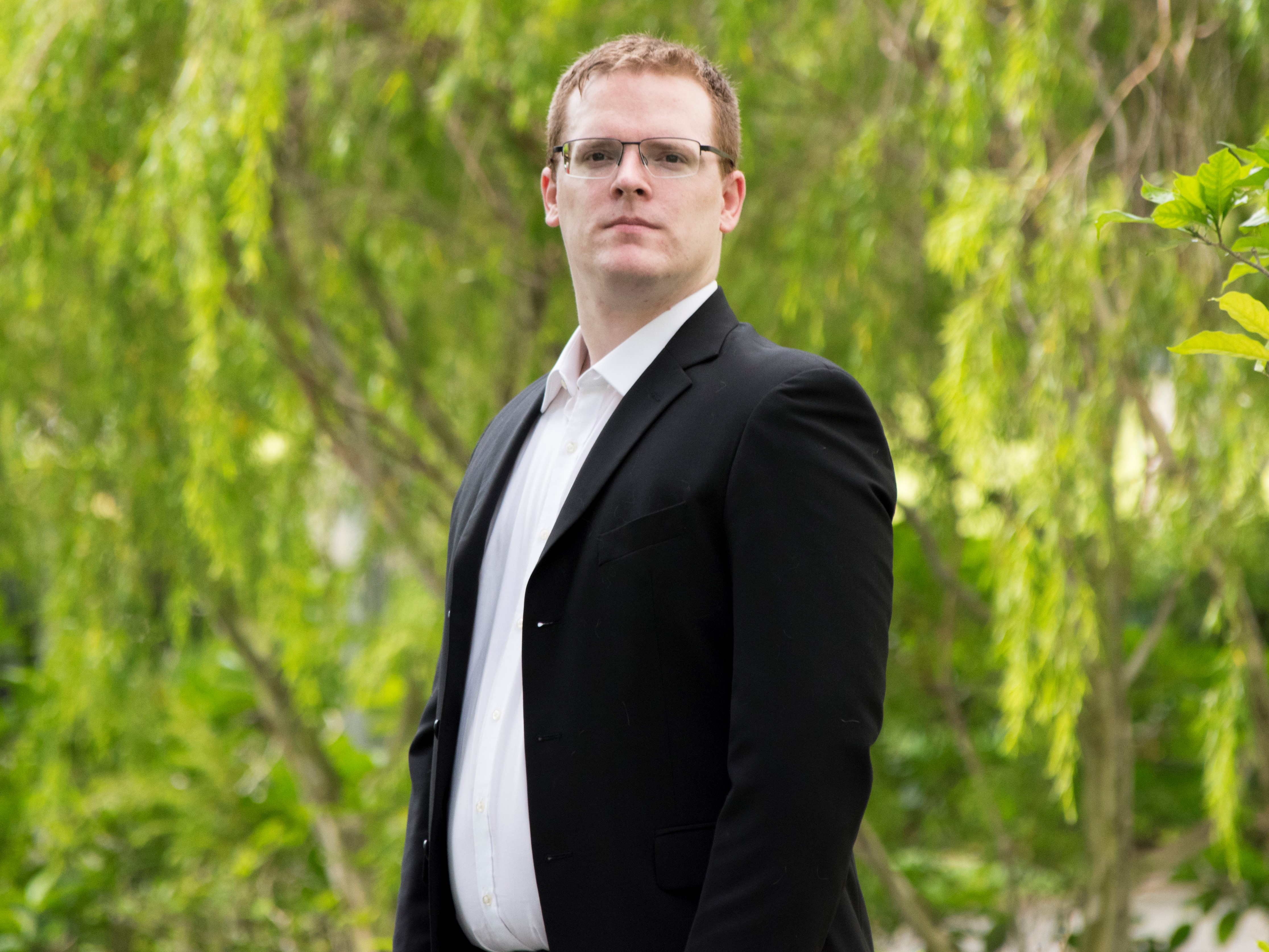
By Jovina Ang
SMU Office of Research & Tech Transfer– There is a shortage of law enforcement agents across the world to handle the wide range of incidents that occur on a day-to-day basis.
This explains why many law enforcement agencies are moving from a reactive to proactive policy as a way for deterring criminal activity.
Proactive policing requires the law enforcement agencies to establish an efficient crime prediction system on one hand, and an effective response system to manage and curtail crime activities on the other hand.
And many law enforcement agencies are moving towards the adoption of data-driven artificial intelligence (AI) to inform their policy strategy.
“Critical to developing an efficient law enforcement system is an intelligent and a fluid scheduling system for law enforcement agents,” Assistant Professor of Computer Science (Practice) Jonathan Chase told the Office of Research & Tech Transfer.
He added: “While there exist such intelligent systems, many of the systems still do not function optimally. Nor are the systems tailored to the demand characteristics of a given day.”
“Executing a daily deployment schedule system is critical for two reasons. One, for the law enforcement agency to adjust to the demand of any given day, and two, to deter criminals from anticipating where the law enforcement agents will be stationed”, he continued.
“A daily deployment system can also enable the commander to do manual adjustments depending on the needs of the day for meeting unanticipated needs, adjusting unforeseen manpower shortage and so on,” he went on.
To build upon previous work on intelligent scheduling and patrol system, Professor Chase and his collaborators, namely, Tran Phong, Kang Long, Tony Le and Professor Hoong Chuin Lau worked with a local law enforcement agency, to generate incidents using machine learning while incorporating real life requirements such as varying manpower, break times, manual pre-allocations and a variety of spatio-temporal demands of a typical law enforcement agent face.
The research
Fundamental to the research that was conducted, was using historical data to simulate the incidents for a data-driven AI system that was on trial with a local law enforcement agency.
The system, which was called the GRAND-VISION (Ground Response Allocation and Deployment – Visualisation, Simulation and Optimisation) system, was implemented in Python and based on the Django framework for database integration and linked to a front-end web-based app.
The research also mimicked the real-life demands mentioned above.
In so doing, the research team was able to develop a patrol system that could generate an hourly deployment schedule of agent resources so that the agency could provide the best response to incidents.
The system that was developed leveraged the use of a generative deep machine learning model for more precise predictions of incidents as it incorporated the use of both the latitude and longitude location coordinates of historical incident data.
The system implemented a dispatch planner that combined an incident generator, an optimiser, a scheduler and a simulator. This helped to design a deployment schedule that could assign agents to patrol locations throughout their shift, thus building fluidity in the patrol system.
Outlook
There are multiple contributions from the research.
In conveying to the ORTT, Professor Chase said: “The system that was developed has been proven to be more intuitive in dispatching agents to the locations where incidents occur as it can intelligently assign the nearest agent.”
“This has a lot of practical implications in ‘correctly’ scheduling law enforcement agents. For example, incorporating flexible break times which is an important component of intelligent scheduling for such a system to function efficiently in the real world. The use of deep machine learning has made accurate predictions in such systems possible. And finally, the simulation combined both historical incident data and incorporated real life requirements of any law enforcement agency including varying manpower,” he elaborated.
This research is funded by the National Research Foundation Singapore under its Corp Lab @ University scheme and Fujitsu Limited as part of the A*STAR-Fujitsu-SMU Urban Computing and Engineering Centre of Excellence.
Back to Research@SMU May 2022 Issue
See More News
Want to see more of SMU Research?
Sign up for Research@SMU e-newslettter to know more about our research and research-related events!
If you would like to remove yourself from all our mailing list, please visit https://eservices.smu.edu.sg/internet/DNC/Default.aspx