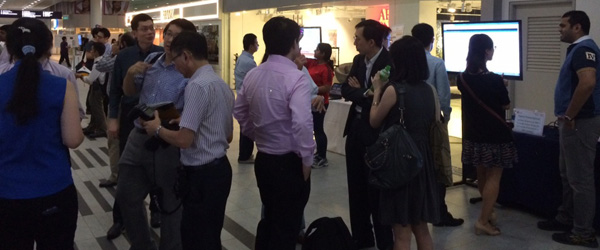
![]() |
![]() |
A brainchild of SMU and Carnegie Mellon University, the Living Analytics Research Centre (LARC) held an exhibition at the SMU Concourse on 26 March 2014. Themed “Better Living Through Analytics”, the exhibition featured its latest research on behavioural and social analytics. The full-day event attracted a steady flow of visitors including LARC’s industry partners, as well as representatives from companies and government agencies such as the National Research Foundation, Economic Development Board, Ministry of Home Affairs, and National Environment Agency.
Bringing together data mining, machine learning, statistics, management, social and behaviourial science, LARC develops new concepts, methods, and tools that are experiment-driven, closed-loop, real-time, and practical. Its primary directive is to: (i) develop analytics methods that discover business and social insights from societal-scale data; (ii) design closed-loop iterative experiments that determine factors affecting consumer and social behavior; and (iii) study the influence of social connectedness on digital media consumption.
Research@SMU casts the spotlight on two innovations that stood out among the impressive lineup of research work and demonstrations showcased:
1. LASER – Simplifying Online Experimentation on Consumer and Social Insights
Authors: Kwan Hui LIM, Ee-Peng LIM, Palakorn ACHANANUPARP, Adrian VU, Agus Trisnajaya KWEE and Feida ZHU
Websites are an essential platform for businesses to communicate information and facilitate online transactions. The need to build websites that attract user attention and promote ease of online purchases has led to a corresponding emphasis on tracking user browsing habits, and measuring the effectiveness of online services and web design.
Although large-scale, online controlled experiments allow organisations to objectively evaluate and optimise website effectiveness, considerable research efforts and programming expertise are needed to perform such experiments. To help businesses conduct customised online experiments with minimal effort and modifications to their existing websites, researchers at LARC have developed the Living AnalyticS ExpeRimentation (LASER) system, which performs the following functions:
i. Simplifying experimentation setup via a Graphical User Interface format
ii. Automating random assignment of participants into control or treatment groups
iii. Determining the exposure of different groups to different web interface variations
iv. Monitoring user activity in these groups
v. Measuring participants’ responses
vi. Visualising real-time results in an interactive dashboard
Through these functions, the LASER system tracks users’ browsing behaviour before and after a change is made to a web design or service, so as to evaluate the observed differences. To demonstrate its application, researchers at LARC used the LASER system to run a series of experiments to examine the effects of an authority message on visitors' browsing behaviour in online announcement sites. The message, which featured a prominent speaker and his achievements, was deliberately introduced to the treatment group whose online activities (mouse clicks and mouseovers – see Figure 1) were tracked.
Figure 1: Heatmap of Mouse-clicks for Control (left) and Treatment (right) Versions
The findings revealed that the presence of an authority message increased the group’s interaction with other elements featured on the webpage. In particular, participants displayed greater interest in reading the speaker’s biography and talk abstract, and checking out peer experts and event details. Similar findings were observed when the experiment was replicated across other seminar-related websites. By extrapolation, this implies that e-commerce sites could also benefit from the use of authority messages to draw consumers’ attention to specific merchandise or product categories.
The potential of LASER was showcased at the 2014 International Conference on World Wide Web in Seoul. By simplifying and automating the design and implementation of large-scale, online experiments, it offers a convenient and scalable platform for organisations to manage various aspects of controlled experiments using online users in real-life application scenarios.
2. Group Detection Analytics for Indoor Public Spaces
Authors: Rijurekha SEN, Youngki LEE, Kasthuri JAYARAJAH, Archan MISRA, Rajesh Krishna BALAN, Siyuan LIU and Ramayya KRISHNAN
Human behaviour is heavily influenced by social context. Whether it is the size of a group that an individual is associated with, or the relationship that defines the collective actions of a group, group-related context affects both individual and collective behaviour. When applied to large, urban spaces such as shopping malls and theme parks, social context has a bearing on the type of stores, attractions and activities that groups patronise, as well as the amount of time spent.
This presents opportunities for marketers who want to understand and influence consumer behaviour. For example, ad-hoc movie promotions would appeal more to a group of three to five young adults than a group of twelve who is more likely to have made an advanced booking. Such data that is derived through the use of analytics can help marketers know how distinct groups in public spaces behave, thus improving their efficacy in targeting timely and relevant promotions and services.
However, group detection in crowded places poses two main challenges. Firstly, individuals in a high-density space often exhibit similar movement patterns inadvertently, regardless of being in a group or not. This rules out proximity as an implicit indicator of relationships. Secondly, location-tracking systems in indoor environments are rare, and they tend to be unreliable under high occupancy and density. To resolve these issues, researchers from SMU’s LiveLabs and LARC have conducted a joint analysis of human movement data collected from three distinct types of public space:
- SMU campus where groups of students and staff recur almost on a daily basis;
- large shopping malls in Singapore and Korea where consumer groups recur mostly on a weekly or monthly basis; and
- a major airport in Asia where groups rarely recur
Due to inherent constraints and differences in group movement characteristics, the venues offered varying degrees of accuracy for group detection, with some providing access to inertial sensing data from consumers’ mobile phones. Researchers from LiveLabs and LARC addressed these differences by tackling the trade-off between accuracy, latency and energy requirements. As a result, two primary classes of group detection technologies were created:
1. Offline Detection
This class of algorithms captures similarities among individuals’ movement traces that are collected over an entire visit episode along these dimensions: (i) the space and time that they occupy; (ii) the semantic boundaries of such spaces; (iii) the transitions between such spaces; and (iv) the velocity of their motion.
By combining similarity measures along these dimensions in a weighted fashion, the proposed algorithms are able to detect groups with accuracies of 75 per cent and higher, even though there are several thousands of people in the indoor space. Although offline detection takes place after an entire visit episode has occurred, it offers the advantage of accurate group detection.
2. Online Detection
In contrast, online detection enables similarities between individuals to be computed in near realtime by tapping on the same space/time information used in the abovementioned offline detection algorithms, as well as mobile sensor-generated data which detects motion-related activities such as walking, turning, standing still, and floor height changes (see Figure 2). Here, detection accuracy and latency are important performance metrics, as researchers seek to identify groups while they are in the middle of visiting these public spaces.
Figure 2: Illustrating the “motion events” used in online group detection
To achieve this, algorithms that use such location and motion-oriented features are developed to achieve high accuracy in group detection (80 per cent of groups were detected within ten minutes of initiating their visits). Such algorithms are highly applicable to places such as malls and airports where groups usually enter, exit and move together in a coordinated fashion over measurable timescales.
By leveraging on context-aware technologies to track group activity and movement patterns, these innovations herald promising options for retailers to identify visitors' in-store preferences, attitudes and intentions. This ultimately enables the provision of context-relevant information and incentives to consumers at the right time, in the right way.
See More News
Want to see more of SMU Research?
Sign up for Research@SMU e-newslettter to know more about our research and research-related events!
If you would like to remove yourself from all our mailing list, please visit https://eservices.smu.edu.sg/internet/DNC/Default.aspx