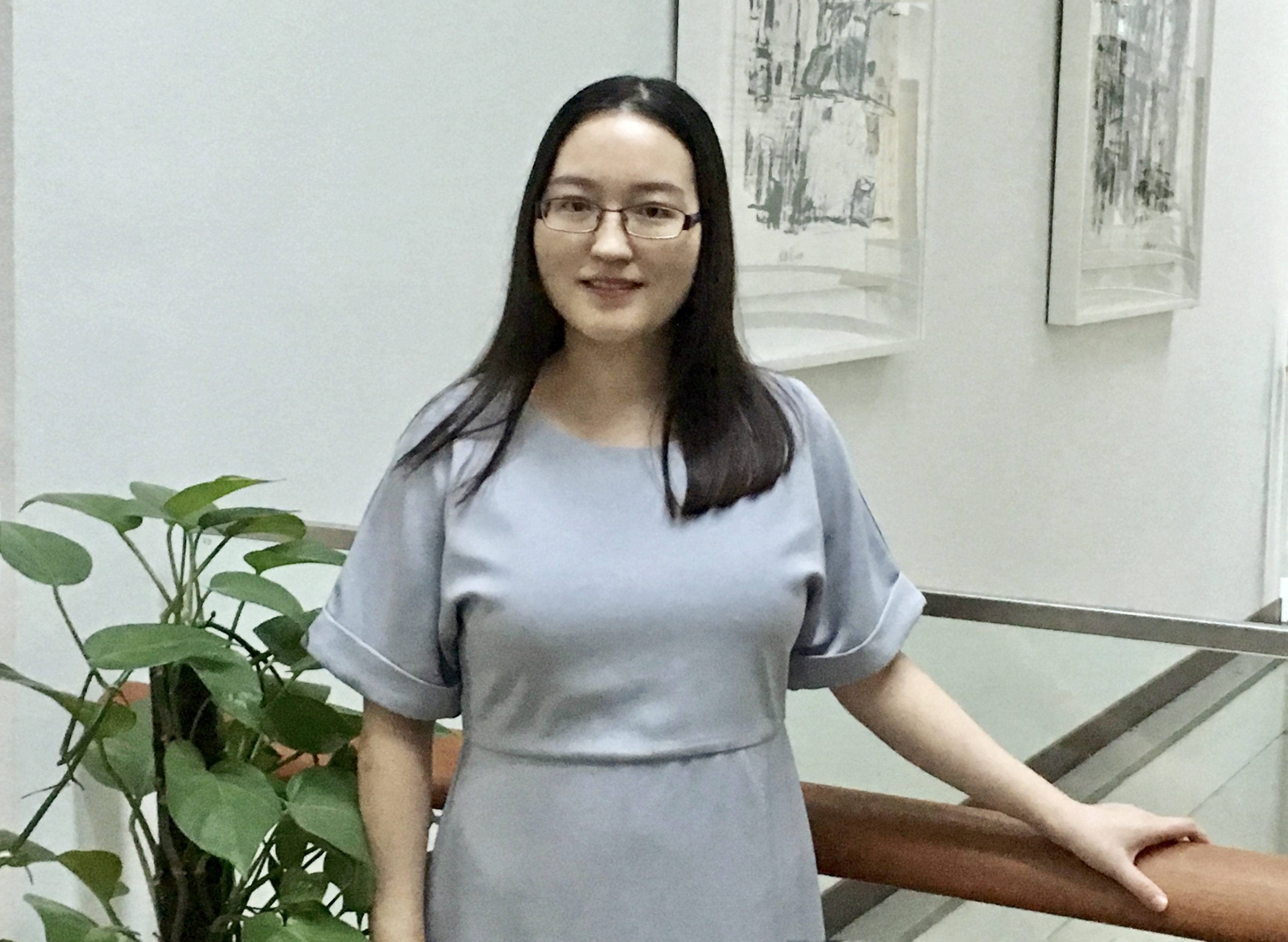
By Cheah Sin Mei
SMU Office of Research & Tech Transfer – Grab, Go-Jek and the now-defunct Uber (in Singapore) are familiar names of ride-hailing services that have shaken up the local taxi industry. An obvious benefit of this disruption is that the man on the street is happy with lower fares, greater convenience without having to flag down taxis on the roadside, as well as cashless payment as he could simply step out of the car without having to dig into his wallet for notes and coins.
But to academics and policy makers in the transport sector, their perspective is often focused on the macro industry level. For example, is ride-hailing more efficient than street-hailing? That is, is the average waiting time for passengers shorter using the ride-hailing system? This was precisely the kind of research questions that SMU Assistant Professor Feng Guiyun is interested to find out.
“There is on-going debate about the two platforms, as to which is more efficient, in terms of how long you need to wait. This research is motivated by my own experience”, said Professor Feng, who holds a double Ph.D. in Industrial and Systems Engineering from University of Minnesota and Management Science from the City University of Hong Kong.
Making better decisions
As a Professor of Operations Management, Professor Feng’s research interests include the sharing economy, revenue management and the use of stochastic modelling to study operational efficiency.
“Operations management is concerned with how to make the operation decisions as effective as possible”, said Professor Feng, who joined the Lee Kong Chian School of Business in January 2019. “As long as decision-making is involved, we can build models to obtain insights and pursue optimal operational decisions”, she added.
In a nutshell, Professor Feng summarised: “I am doing stochastic modelling and analysis in operations management which has multiple applications in areas like revenue management, where I work on discrete choice modelling, and another area is sharing economy, where I focus on ride-sharing.”
Ride versus street-hailing: Which is more efficient?
In an article titled ‘We Are on the Way: Analysis of On-Demand Ride-Hailing Systems’ published in the book Sharing Economy: Making Supply Meet Demand, Professor Feng and her fellow researchers discovered that the on-demand ride-hailing system is not always more efficient than the traditional street-hailing mechanism. Upon comparing the two modes of taxi, Professor Feng further determined three conditions under which ride-hailing outperformed street-hailing.
First, the ride-hailing system is superior to street-hailing if the distance between a private-hire driver and the pick-up location of his passenger is moderately short. When a driver has to travel a long distance to fetch a passenger, he may miss the chance to serve another customer who is at a closer distance. This scenario may have an adverse effect on an impatient passenger, as the longer the waiting time, the higher the tendency to cancel the booking. Passenger abandonment ultimately leads to lower utilisation of the system (i.e. fewer passengers can get a cab) and longer average waiting times for all passengers.
Second, the ride-hailing system achieves high efficiency when the customer demand for taxis is very high (i.e. many waiting passengers) or very low (i.e. many idle taxis). This is because the system can optimise the matching of a driver-passenger pair by choosing the shortest distance between them.
Third, in a city with a complex street grid network that is difficult for the driver to navigate, a passenger who books a cab via a taxi mobile app will get his ride sooner than if he were to try his luck on the streets. Thanks to the efficiency of the booking app algorithm, the private-hire driver is able to locate the nearest passenger more quickly as compared to another driver plying the streets.
When classic theory is inadequate
While classic queuing theory was previously used to resolve similar on-demand matching of drivers and passengers, it did not take into consideration the en route time, that is, the time spent to travel to a passenger’s pick-up point.
To overcome the inadequacy of classical theories, Professor Feng and her team proposed an approximate queueing model to capture the en route time, and also imposed a distance limit when matching the driver and the passenger. In addition, to reduce the average waiting time, they also imposed a distance limit when matching the driver and the passenger, and derived a heuristic method to determine a near-optimal distance cap.
Discrete choice models
The decision of whether to hail a taxi on the street or book via an app is a consumer’s choice. One way in which researchers studied how people made choices is through discrete choice models – a modelling method that explains and predicts the option an individual chooses among a finite number of alternatives.
Professor Feng’s second area of research interest is in discrete choice models, and she has published two papers that contributed to this body of knowledge. The first article - ‘On the Relation between Several Discrete Choice Models’ published in the Operations Research Journal, she examined the relationship between the random utility model, the representative agent model and the semi-parametric choice model. From a new perspective using a welfare-based approach, she established the similarities between the models given the number of available alternatives.
In her second article titled ‘On Substitutability and Complementarity in Discrete Choice Models’ published in the Operations Research Letters Journal, her team studied how alternatives within a set of options could substitute and complement one another. “These models are useful in demand forecasting”, said Professor Feng, and she highlighted its application in retail contexts such as flight ticketing, grocery and fashion retailing.
As stochastic choice models can be widely applied to several contexts including transport economy and information systems, Professor Feng is also keen to collaborate with researchers in these disciplines who may be interested to work together.
Back to Research@SMU May 2019 Issue
See More News
Want to see more of SMU Research?
Sign up for Research@SMU e-newslettter to know more about our research and research-related events!
If you would like to remove yourself from all our mailing list, please visit https://eservices.smu.edu.sg/internet/DNC/Default.aspx