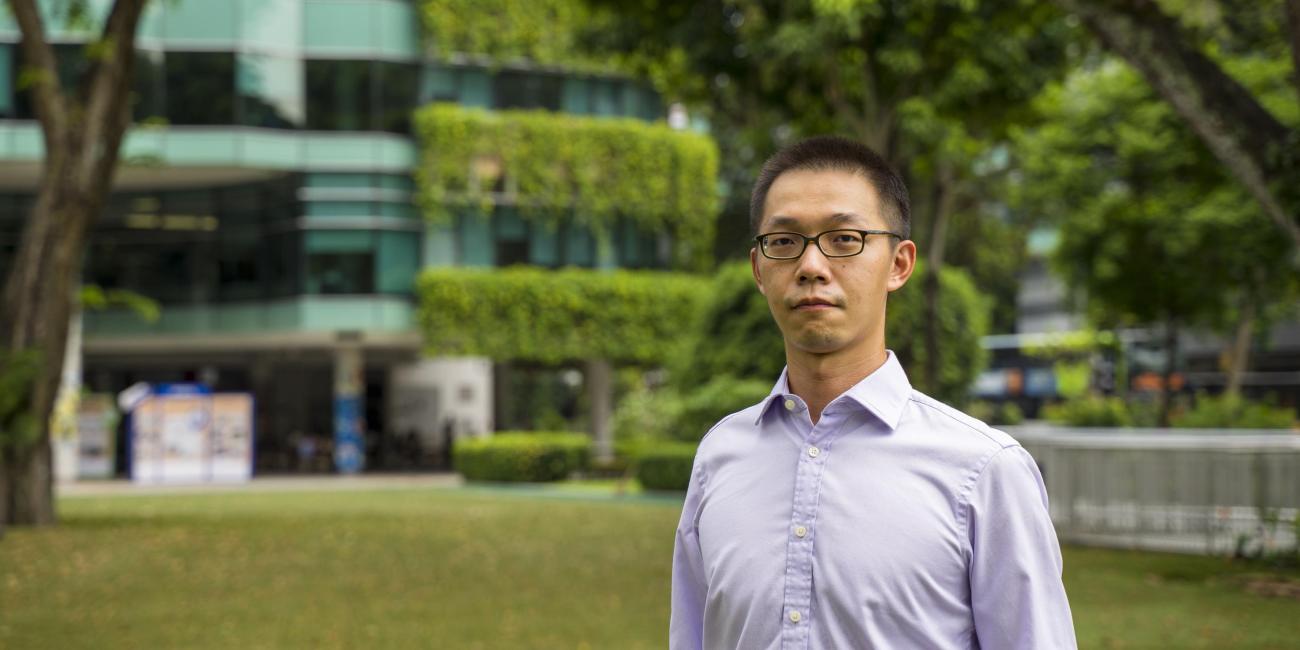
Spatial crowdsourcing, which consists of location-specific tasks that require people to be at specific locations physically to complete them, has stimulated various new applications such as taxi hailing and food delivery.
A key enabler for these spatial crowdsourcing-based applications is to plan routes for crowd workers to execute tasks, given the diverse requirements of workers and the spatial crowdsourcing platform. Take on-demand taxi-dispatching, a common spatial crowdsourcing application, as an example. There are three parties involved: the platform (e.g., Grab), workers (e.g., Grab taxi drivers), and tasks (e.g., taxi requests submitted by Grab passengers). The platform needs to develop route plans for each driver to pick up as many passengers as possible, so as to maximize the platform’s revenue; at the same time, it has to ensure the location privacy of passengers.
Despite extensive studies on task planning in spatial crowdsourcing, few have accounted for the location privacy of tasks which imposes an extra constraint on task planning, making the problem challenging.
To tackle this challenge, Assistant Professor Zhou Zimu of SMU School of Computing and Information Systems and his co-authors undertook research and submitted a paper titled, “A Differentially Private Task Planning Framework for Spatial Crowdsourcing” last year. It won the Best Paper Award at the 22nd IEEE International Conference on Mobile Data Management (MDM2021). IEEE is the world's largest technical professional organization dedicated to advancing technology for the benefit of humanity.
Prof Zhou said, “The pandemic has accelerated the digitalization of everyday life, including mobility data. While embracing the convenience brought by various digital applications and services, we also need to care about delivering these applications and services in a privacy-preserving manner. I am delighted that our work towards this direction was acknowledged by MDM 2021, a premier international conference on mobile data management.”
On why it is important to have location privacy of tasks as a platform already knows the location of a task when it receives and sends out a customer request to taxi drivers or food delivery riders, Prof Zhou replied that in most spatial crowdsourcing applications in use, a customer will reveal his/her location to the platform. “This is because we assume the platform is trustworthy and will not misuse the location data. However, there are growing concerns about data privacy. Many countries and regions have also launched laws and regulations to enhance user data privacy.”
He added that since applications such as taxi-hailing and food delivery are frequently used in everyday life, an untrustworthy platform may extract personal mobility patterns from these location records. For example, the platform may easily infer one’s workplace and home address, which may further reveal one’s profession and income level, if combined with other information. Such information could be misused for unwanted location-based advertisements and scams, among others.
The solution put forward by Prof Zhou and his team is Geo-Indistinguishability, a common differential privacy metric for location-based systems to assess the location privacy of tasks. This metric protects the location information within a radius and the privacy level is dependent on the radius. For example, by ensuring a given level of Geo-Indistinguishability, the platform may tell whether the request for taxi came from Singapore or Johor, but it cannot distinguish whether the taxi request is from SMU or Gardens by the Bay.
“To protect the locations of tasks, we obfuscate the original task locations following the planar Laplacian distribution. This mechanism ensures geo-indistinguishability of the task locations. To make efficient and effective task planning with obfuscated locations, the team designed a dynamic programming based planning algorithm that accounts for the long-term utility (i.e. revenue generation) when inserting a task into a worker’s route plan. It is also worth mentioning that another contribution of our work is a theoretical analysis on the impact of privacy protection on the effectiveness of task planning,” added Prof Zhou.
On the benefits of the solution, Prof Zhou shared that it allows spatial crowdsourcing platforms to perform effective task planning while protecting location privacy of tasks. “This benefits both the customers and the platform. From the customers’ perspective, since the platform is unaware of their locations, they do not need to worry about misuse or leakage of their location information, which may reveal their daily mobility habits. From the platform’s perspective, it can still achieve high revenue without knowing the true locations of tasks. Furthermore, it can advertise its privacy-preserving task planning strategy, which may attract more customers.”
Looking ahead, Prof Zhou intends to continue his research in this domain, as awareness of privacy and security in spatial crowdsourcing is becoming increasingly important. “Task planning is only one common operation in spatial crowdsourcing. There are other operations such as spatial queries, quality control, and data mining on spatial data, to name a few. It is an interesting research domain to provide privacy or security guarantees while achieving high effectiveness and efficiency in these operations,” he enthused.
Prof Zhou’s research also includes embedded and mobile intelligence. His vision is to integrate sensing, computing and decision-making into everyday IoT infrastructures for intelligent individual and urban services. Some of his on-going research topics include how to deploy deep neural networks on resource-constrained platforms such as a smartphone or a smartwatch, and how to exploit big data to improve the efficiency of large-scale sensing systems such as for air pollution monitoring.
Originally published at https://news.smu.edu.sg/news/2022/03/24/planning-tasks-effectively-while-preserving-their-location-privacy-spatial
Back to Research@SMU May 2022 Issue
See More News
Want to see more of SMU Research?
Sign up for Research@SMU e-newslettter to know more about our research and research-related events!
If you would like to remove yourself from all our mailing list, please visit https://eservices.smu.edu.sg/internet/DNC/Default.aspx