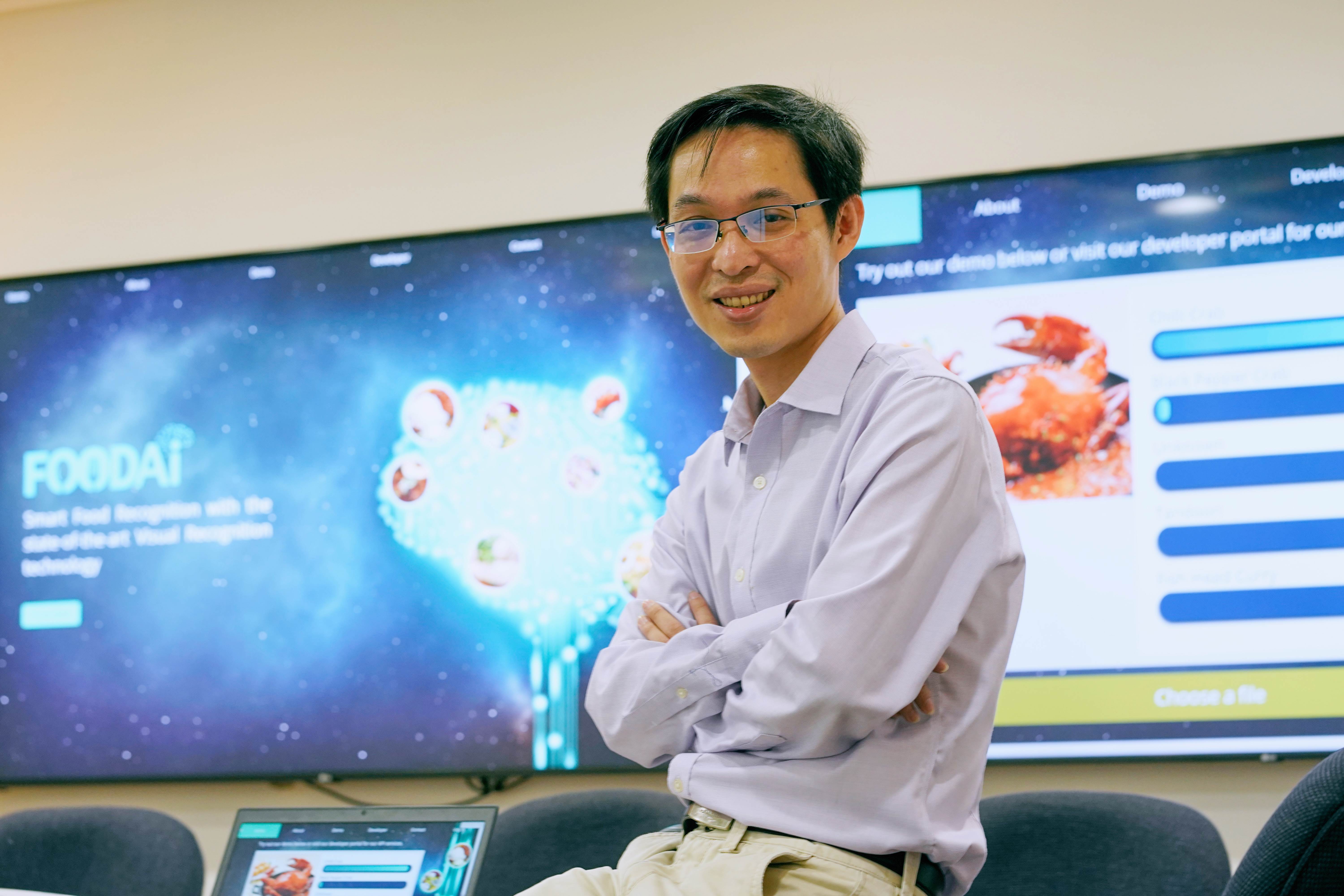
By Jeremy Chan
SMU Office of Research & Tech Transfer – As a tool for information processing and problem solving, the human brain is unparalleled in its competency. While in recent years artificial intelligence (AI) has emerged to mimic certain aspects of human intelligence, getting AI to a level of performance that rivals the human brain is no simple task.
Reaching this milestone will take effort on the part of researchers like Associate Professor Steven Hoi of the Singapore Management University (SMU) School of Information Systems, who are exposing the limitations of current machine learning methods and ‘tutoring’ AI to make it smarter.
“I have been doing research on machine learning and AI for more than 15 years,” says Professor Hoi. “Specifically, my recent research addresses pressing and open challenges of machine learning and deep learning to effectively solve a range of real-world problems in AI and big data analytics.”
Continuous learning for machines
Professor Hoi’s interest in machine learning was sparked when he was a graduate student at the Chinese University of Hong Kong. Working on the problem of automatic search and retrieval of images from large databases, he realised that machine learning could be immensely useful for refining the accuracy of such content-based image retrieval systems.
But beyond the practical applications of machine learning techniques, Professor Hoi is also fascinated with the fundamental science underlying machine learning and AI, essentially asking the question: how do machines learn?
A child learns naturally and continuously when exposed to stimuli from his or her environment, even making inferences from incomplete information. Most existing machine learning techniques, however, need to be trained on large, well-labelled datasets before they are capable of performing a specific function, such as to caption an image, says Professor Hoi.
“The limitation of current machine learning methodologies is that they are static. They do not evolve and adapt to an endless, continuous stream of data,” he explains. This is analogous to a student studying from a large volume of textbooks before entering into an examination hall. Should there be new problems presented, the student flounders and may not get a good grade.
What Professor Hoi seeks to do is to make machines capable of online learning. This means that the learning algorithm must be able to consistently update its predictive function using information that is fed to it sequentially rather than as a complete batch. He has developed numerous online learning algorithms over the years, experimenting with different statistical and classification techniques and evaluating their effectiveness.
Furthermore, recognising the need for a centralised database of online learning techniques, Professor Hoi established LIBOL, an open-source library of online learning algorithms and their variants, which allows researchers to make side-by-side comparisons of available algorithms and explore their applications. He announced the launch of the platform in the Journal of Machine Learning Research in 2014, and continues to curate and update the library with important findings in his field.
Teach less, learn more
Another limitation of current machine learning techniques is their reliance on large datasets that are costly both in terms of time and money to obtain. Hence, Professor Hoi is investigating ways to develop algorithms that make meaningful predictions using a limited number of data points.
He is also looking into one-shot learning and transfer learning, wherein the knowledge gained by the machine from the analysis of one training dataset in one domain is stored and used as a starting point for solving a related problem with few data points in another domain. This would greatly increase the versatility of machine learning and reduce the level of human supervision of AI.
The multi-pronged approach that Professor Hoi adopts is necessary because “in practice, no single learning algorithm can win for all scenarios”, he says. “As a researcher, it is important to understand the fundamentals of each family of machine learning techniques and be aware of their advantages and disadvantages under different situations and contexts,” he emphasises.
Bridging theory and practice
But beyond trying to understand the fundamentals of machine learning, Professor Hoi also develops applications that make use of smart algorithms to perform interesting functions and solve real-world problems.
“For example, one of our recent projects is FoodAI. We aim to build food image recognition technology for smart food logging applications. Deep learning is applied to train image recognition models to automatically identify and caption a food photo captured by a user via a mobile app. The application can further report the nutritional information of the food from the photo to help users track and analyse their eating behaviours,” he says.
Additionally, on the cybersecurity front, Professor Hoi has developed an AI-enabled solution called AntiMalweb, which can be used to detect malicious URLs on the internet. Online learning and deep learning algorithms, among others, are used to automatically flag suspicious websites to protect internet users from phishing attacks.
Striking a balance
Given the multifaceted nature of machine learning and AI research, Professor Hoi strives to divide his time between theoretical and practical pursuits.
“Typically, theoretical research is more likely to yield long-term impact, while practical research is more translatable and easier to observe in the short term. I always try to include both in my research agenda to achieve a good balance,” he says.
Another balance that needs to be achieved is that between AI and society. Professor Hoi thinks that rather than threaten human primacy, AI will augment our abilities and function as our ally in the future. But until then, AI still relies on humans to help it become more intelligent.
“The long-term vision of my research is to create intelligent machines that empower humans in tackling various real-world problems,” says Professor Hoi.
Back to Research@SMU Issue 57
See More News
Want to see more of SMU Research?
Sign up for Research@SMU e-newslettter to know more about our research and research-related events!
If you would like to remove yourself from all our mailing list, please visit https://eservices.smu.edu.sg/internet/DNC/Default.aspx