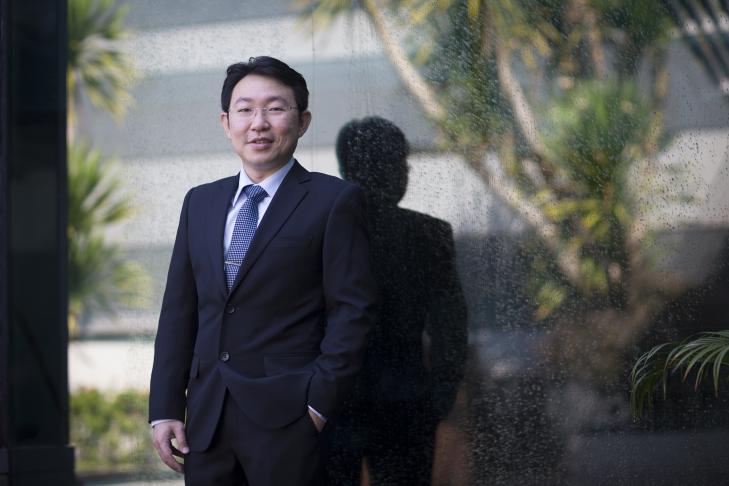
For Assistant Professor Hady Wirawan Lauw, data mining is not just about numbers; It is premised on human behaviour, and the multitude of decisions that we make every day.
By Chin Wei Lien & Vicki Yang
SMU Office of Research – Data mining is one of the most ubiquitous business tools on the market today. The ability to convert raw data into useful information is responsible for the success of some of the biggest companies in the world, such as Amazon, Google and Facebook.
E-commerce and social media sites, however, are not the only ones on the data-mining wagon. Other businesses, from banks to supermarket chains, are utilising data mining to understand their customers better, and in turn, maximise their profits. For instance, from the placement of products to the arrangement of shelves in a supermarket, every detail has been carefully analysed, calibrated and executed based on data collected on patrons’ purchasing behaviour over the years.
Identifying useful patterns from large amounts of information — or finding order in the madness — is a key area of investigation for Assistant Professor Hady Wirawan Lauw from Singapore Management University’s (SMU) School of Information Systems (SIS).
Deep dive into data
Data mining is most commonly associated with the study of numbers and figures. Some see it as a way for businesses to personalise services, improve consumer experience and generate more revenue. However, researchers such as Professor Lauw are discovering that uncovering data on people as individuals or as a community is not quite enough. “When people used to talk about data mining, they’d talk about a lot of data, such as weather data or supermarket data: it used to describe the world itself,” he explains. “As we go further, we realise that the world remains the same — but what is different are the users.”
So what is changing? Professor Lauw believes that with greater access to services such as social media, users are generating more data than ever before – either consciously or subconsciously. “When you watch television, you’re watching what everyone else is watching. The only control you have is the remote control and which channel you watch. In reality, when people are watching television, there is a lot of information coming in. If you can track when they switch channels, and what kind of programmes are actually playing, we can tailor better services to these users.”
With more people using online resources which generates more data as a result, there also needs to be a better way for researchers to sift through all the data and recommend more efficient ways to convert them into real-world results — something that Professor Lauw is working on.
More granular recommendations
During the 2014 International World Wide Web Conference Committee in Seoul, Professor Lauw together with his PhD student presented a generative model from their paper, Modelling Contextual Agreement in Preferences, aimed at providing a more granular and targeted approach to product and service recommendations.
For example, it is common for e-commerce sites to recommend other products or services to users based on their search or purchase history. The issue with many of such recommendation systems, however, is that they often cast too wide a net, resulting in inaccurate predictions of what the users want.
To overcome that, Professor Lauw’s generative model, known as the Differential Probabilistic Matrix Factorization (DPMF), takes into account the probability of agreement between two users within different contexts. “Friends agree a lot, but not all the time; it’s important to know those instances when you disagree. Understanding when people are similar and different is going to give us a bigger picture,” explains Professor Lauw.
In the real world, this model may have a huge impact on business decisions and consumer choice. For example, by applying the model to raw data, businesses would be able to present more precise recommendations to users, which in turn could have a positive effect in consumer purchasing, thus translating into revenue for the business.
Addressing service gaps
Aside from financial institutions and technology giants, companies from other sectors — telecommunications, insurance, healthcare, public transport, retail and direct marketing — are also seeing real-world results from data mining, Professor Lauw notes.
The cable television industry was the focus of his other two papers. The first paper, Mining Revenue-Maximising Bundling Configuration, investigated consumer preferences for bundling of television programmes and determined optimal bundle configuration to maximise revenue for up to two items. While configuring three or more items involves more complexity, Professor Lauw and his collaborators were also able to derive a set of algorithms that allowed researchers to construct the optimal bundling solution in a way faster than traditional methods.
The second paper, Modeling Preferences with Availability Constraints, co-written with a colleague from SIS, focused on the effect of availability constraint on users. ‘Availability constraint’ refers to the restriction of choices that users make when there are limited options, such as the choice of television channels in a given cable bundle. By predicting the hidden or latent interests of consumers — in this case, the television channels people would pick or not pick if they had unlimited choices — and incorporating these into a model, both consumers and businesses could ultimately enjoy more varied and profitable cable television bundles.
Professor Lauw warns that restricting choices may ultimately mean restricting our understanding of consumer choices. “When you force customers to subscribe to certain channels, you are losing something more valuable than what you gain in sales — you lose information on what people would have done, had they been given access to everything and been allowed to express their preferences freely.”
The wisdom of crowds
What do these models mean for companies and the average consumer? For businesses big or small, adopting these models could mean more targeted data mining solutions, and in turn, a higher degree of personalisation for their consumers. For instance, if a start-up e-commerce site were to adopt the DPMF model, it could configure a more effective method of offering product and service recommendations on their site.
Moreover, such models can apply across different industries. For instance, travel agencies could determine the best way to offer their services using the model, such as offering fully guided tour packages, or bundling free and easy tour packages with optional guide tours for different regions. A hospital can design a series of health screening bundles catered to different age groups, to maximise their revenues in the long run. For the everyday customer, this could mean better recommendations, more varied selections, and better tailored products and services that suit their individual preferences.
Data mining operates on the rationale that perceivable similarities in human behaviour can actually reveal differences which form insights that benefit both consumers and businesses. Ultimately, it all boils down to new ways of discovering hidden preferences based on people’s behaviours, a process that continues to fascinate Professor Lauw.
“We are always faced with too many choices. What data mining can do is to simplify the choice conundrum, and reduce our options to the one that matters the most. Understanding the ‘genome’ of human preferences is key to revolutionising the personalisation of online applications. So in that sense, we can reshape the world,” he shares.
See More News
Want to see more of SMU Research?
Sign up for Research@SMU e-newslettter to know more about our research and research-related events!
If you would like to remove yourself from all our mailing list, please visit https://eservices.smu.edu.sg/internet/DNC/Default.aspx