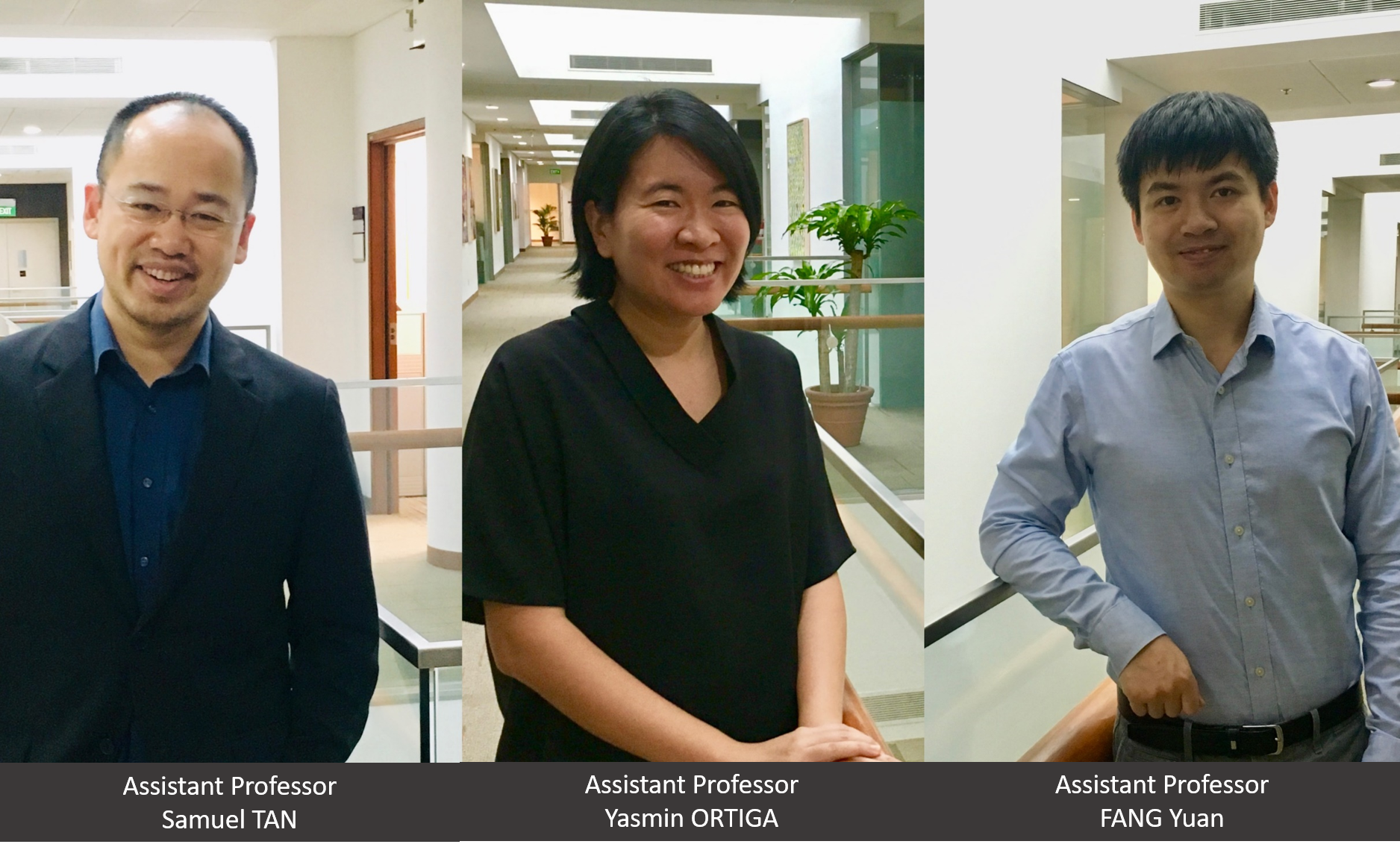
Samuel TAN, Assistant Professor of Accounting
Joined SMU in July 2018
What are your main areas of research and teaching? What business and/or social issues do they address?
I teach and research financial accounting, which concerns the flow of information from firms to external decision-makers such as shareholders and the public. For example, companies provide information to the public in order to get financing, and current shareholders need to be informed about the performance of the firm. I view financial accounting as one of the most important areas of the business world. On the teaching side, I see it as an important service to society to teach the next generation of accountants.
Please explain “Network Analysis” and “Textual Analysis”.
What has been happening in financial accounting research worldwide is a shift towards more sophisticated tools to better understand the business world. These include network analysis and textual analysis. Textual analysis is basically analyzing text. For example, we write programs to parse out text in order to analyse what was written.
As for network analysis, it’s basically analyzing networks. Companies can be seen as nodes in a network, linked to one another. These links could take various forms, such as companies sharing managers or directors. Such links between companies could be relevant in the accounting context because accounting practices can spread between companies, and that includes bad accounting practices.
As researchers and policymakers we want to know how bad or good practices spread, how we can encourage good practices and discourage the spread of bad practices.
When you say textual analysis, are these programs and software supposed to read annual reports? What text are we talking about that serves as input for textual analysis?
Recent research has done this in many different ways. One method has been to look at companies’ annual reports, in particular at the sections where managers have more discretion to write what they wish. For example, managers could try to frame the accounting numbers in a way that makes the firm looks better.
One example is the Management Discussion and Analysis (MD&A) section of the annual report. Some of the research in this area conducts textual analysis of the MD&A across thousands of companies to understand how managers are writing it and how users respond to what was written. That’s one way researchers have applied textual analysis.
Another interesting method I’ve seen in recent papers is to look not just at what managers write, but also at what they say. Managers explain their financial results to shareholders in earnings calls, and the papers explored the possibility of identifying misreporting by the firm based on linguistic analysis of the conference calls. That’s another way of applying textual analysis.
Some studies claim that accountants will be made obsolete by machine learning. Do skills like textual analysis future-proof accounting students?
At some level we’ll still need people to do things. Even with automation, we’ll need people to write and check the programs in the first place, for example. And I don’t think the profession will be completely automated. But students should definitely be aware of how to use all these tools available today.
Also, employers will increasingly be looking for students who know how to write code and who understand modern tools like machine learning. It will definitely be important. As for how fast and how widespread the use of these tools will be, I’m not sure. But I think it’s part of our service to society to make sure that our students will be at the forefront of industry changes.
You are an SMU graduate. What’s it like teaching at SMU instead of being a student? And do you have any pet peeves regarding students’ behaviour in class?
It feels a little weird to be a faculty member because many of my professors when I was an undergraduate here are now my colleagues. But it’s very rewarding because it feels like I’m contributing back to SMU, an institution that contributed a lot to me.
As for pet peeves, I really don’t mind things like using computers in class because I used to do that as a student. I understand the urge to have some distraction or the need to look up something online during the lesson. It may be a function of how interesting the professor can make the class.
Is the onus on the professor to make it interesting? Or is it on the student to make sure he or she is following what’s being taught?
That’s a tough question. It probably goes both ways but I lean a little more on the professor needing to make the lesson interesting, maybe because I’m coming from the perspective of being an SMU student.
Yasmin ORTIGA, Assistant Professor of Sociology
Joined SMU in August 2018
What is your research about?
I do work at the intersection of international migration studies and higher education and globalisation studies. I am interested in how ideas about skills and knowledge shape the way people plan their migration, aspiration and dreams. I also look at how those dreams and aspirations end up affecting the schools that are tasked with offering the skills that will help people move.
I grew up in the Philippines, which is a huge labour exporting country. Growing up, I experienced the strange phenomenon of universities adjusting to labour demands not within the country but to labour demands in countries that were far, far away. That’s how I became interested in the work I do.
You once wrote: “My research investigates how well higher education institutions [in migrant-sending countries] are actually able to fulfil their role as ‘producers’ in the global exchange of migrant labour and what problematic outcomes result from their attempts to do so.” What are the problematic outcomes?
In the case of the Philippines, the higher education system is 80 percent private. What’s happened is they try to behave a little like global factories and anticipate what kind of labour they think will be in demand overseas. They quickly change school structures or offered certain majors in line with that demand. The problems come from not being able to really anticipate what will be in demand four years later.
A recent example is nursing. When you think of the Philippines, you think of the brain drain, that all the nurses have left. But we’ve reached a situation where we actually have 200,000 unemployed nurses in the country because the schools have overproduced nurses. In the end, the local hospitals are unable to absorb them. That’s one problematic outcome.
You also wrote that countries such as Vietnam and China are using the Philippines as a role model on educating for export. What should governments be aware of when pursuing such as strategy?
They should be aware that it’s really difficult to educate people for export, especially if you are talking about higher education. There are the short-term vocational courses that the Philippines does as well, but the history of higher education is about producing leaders of the nation. That’s why it’s seen as such a privilege, and schools should not forget that in this drive to create employable workers.
Vietnam, China, and now I hear some countries in Africa, are looking to bring in higher education into migration programmes. I think that this cheapens the purpose of the university and there is a real risk of turning universities into expensive training centres that simply produce employees for the market.
Should these governments be thinking of vocational institutes instead of higher education then?
The problem is that if you focus on technical vocation, it’s as if you are just producing middle- or low-skilled migrants. The idea is [that] if we have a higher education system that is driven towards global opportunities, we are educating young people who can pursue careers in their own terms. Instead, the Philippines is now treating its own students like commodities, meant to be “produced” then “exported” to foreign employers.
My argument is that you don’t have to completely change the system in order to produce good graduates. One thing that institutions in Singapore are doing very well is focusing on 21st century skills or life skills. The success of your graduate isn’t about running machines that exist in hospitals in Dubai, it’s about being able to think on your feet and dealing with different situations. The problem with educating for export is that it narrows the education system into a vocational role when the purpose of the university was to create people who can think more broadly and generally in the first place.
What would you say to a business student to convince him or her the need to take a social science class? Do they need to? Should they?
I like to go back to the question of: What are schools for? One thing I always tell students is that the purpose of coming to university is not just to prepare for a job; it’s to prepare you to become better people and members of the community. I always believe no matter your course or your major or your ideal job in the future, you’re still a citizen of this world. Whatever social issue happens will still affect you in some way. Social sciences is one way of being able to deal with that and understand it better and develop an opinion.
Do you have any pet peeves about students?
I made the mistake of assuming that students at SMU, a business school, don’t care much about social issues of education and migration. After teaching one course here, I find that they are really engaged and excited about the topic. I realised I’ve stereotyped the students and I shouldn’t have done that.
Pet peeves in general, it’s about the phones. I think the students often don’t realise how often they tend to look at their phones in class, and that’s a pet peeve I share with many professors.
Another pet peeve is when students say that the debates over theory and social issues are “fluffy.” I think they are implying that these discussions don’t have much substance because there is no consensus and sociologists like to say that a lot of institutions that run our lives are only socially constructed. That is partly true. However, I often worry that students who make these comments come away also thinking that such “fluff” is meaningless… when actually, they can mean so much.
As a mother of two in a demanding field, how do you have enough energy to manage everything?
Being a parent makes you more efficient. You suddenly appreciate the time you have. On one level I feel that I do things more quickly, and I feel like I have to make the most of these few hours that I have before the kids wake up.
At the same time, I feel the difference. Academia is tough, but being a parent in academia is a whole different ball game. It helps to have supportive peers, and here at SMU I feel that the environment is very supportive. Students are also surprisingly understanding. When I was single, I could memorise a hundred student names by Week 5. Now, I feel like my brain is full all the time. In my first SMU class last semester, I called one student the wrong name until Week 11.
FANG Yuan, Assistant Professor of Information Systems
Joined SMU in July 2018
What are your main areas of research? How does it impact the business world?
Broadly speaking I’m working on data mining and machine learning. That’s basically trying to find patterns from historical data, and based on these patterns we can make better decisions and also try to predict the future. Because data science and machine learning are very broad areas, I’m specifically working on network data analytics.
What is network data? In traditional data you can think of each data point as an independent data point. In network data we have different entities interacting with each other. For example, we can have different people interacting with each other in a social network. People can talk to each other, they leave messages for each other, they post photos and share them with each other.
We can also have interactions between companies and organisations. It can be a financial network, such as which company has acquired which other company and the shareholders of a certain company. These are interactions between companies. It’s a kind of network data.
Can you explain how what you do can be used to address the areas you just mentioned?
For social networks, people can study the pattern of how people interact with each other, such as how news and information spread. This could add value for marketing purposes and even social sciences studies. Another example would be company networks, especially in the financial industry, where you can see who is interacting with whom and the implications of such interactions.
You worked in a bank in the audit department. How does your area of expertise add value to financial institutions?
Let me talk about the audit department first. It’s basically a risk-control department, trying to contain risk. Traditionally, audit’s role is to identify risk and then to mitigate it. With data science, it’s now possible to be one step ahead and predict potential risks.
There’s lots of network data in everyday life including within financial institutions. By looking at data, financial institutions can catch risks before they happen.
By risks do you mean bad loans? Identifying high-risk borrower profiles?
That’s partly true. If you have a data science model, you can give some kind of score to any given borrower but it should only be used as guidance instead of a direct outcome. At the moment I believe domain experts are still needed but they can use what has been discovered in the data as reference. This can accelerate the approval process but not necessarily replace it.
It’s not just limited to assessing risks in loans. It can also be applied to risks such as erratic employee behaviour, or risks in financial markets and not just individual borrowers.
What misconceptions does the general public have about data scientists such as yourself?
One way people misunderstand data science and machine learning is that it is some sort of magic where you throw some numbers into a model and you get an outcome. Actually it’s not really magic because you can’t collect some random data and expect it to work.
You need two criteria. One, you need a large amount of data, not just in terms of volume but also time span, preferably many years instead of just months. You also need quality data. If you apply one methodology while collecting one year’s data, and then you apply a different methodology for another year’s data, the data quality would be bad and you cannot expect anything good from the data science model.
Why should a non-Information Systems student take up your classes?
In today’s world we have more and more data. 20 or 30 years ago, before the internet age, there wasn’t such a large amount of data available. Now with cheaper storage and lower equipment costs, almost every organisation is collecting and storing larger amounts of data. In almost every field we can make better use of such data.
Even for non-information systems students, such as those working in finance, they can still use data in their financial work to make better decisions by gaining insights from historical patterns. Student in social sciences, who traditionally conduct small scale surveys, now have social network platforms. They can make use of the data made available by these social networks to conduct large scale studies, but with issues of privacy in mind to only work on properly anonymised data with user consent.
What’s the new exciting field in your area of expertise?
People are talking about deep learning, which has had considerable success in other data forms such as images, videos or even text. There are also trends to have deep neural networks in network data. People are starting to apply deep learning to network data, and not just regular network data but so-called heterogeneous network data.
How do you explain deep learning to someone who is not a data scientist like yourself?
It is basically a mathematical model but it mimics a neural network. It is, in essence, an artificial neural network that mimics that of a brain. It has different layers where each layer is like a mathematical formula. We go from the first layer to the last layer which produces the output or predictions. The first layer handles the input - it is a simulation of what biologists believe the brain is doing [when it receives information]. The data that goes into the first layer affects what comes out of the last layer.
Back to Research@SMU February 2019 Issue
See More News
Want to see more of SMU Research?
Sign up for Research@SMU e-newslettter to know more about our research and research-related events!
If you would like to remove yourself from all our mailing list, please visit https://eservices.smu.edu.sg/internet/DNC/Default.aspx